Exploring Text Mining Across Industries and Contexts
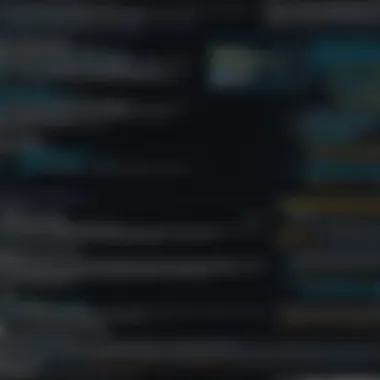
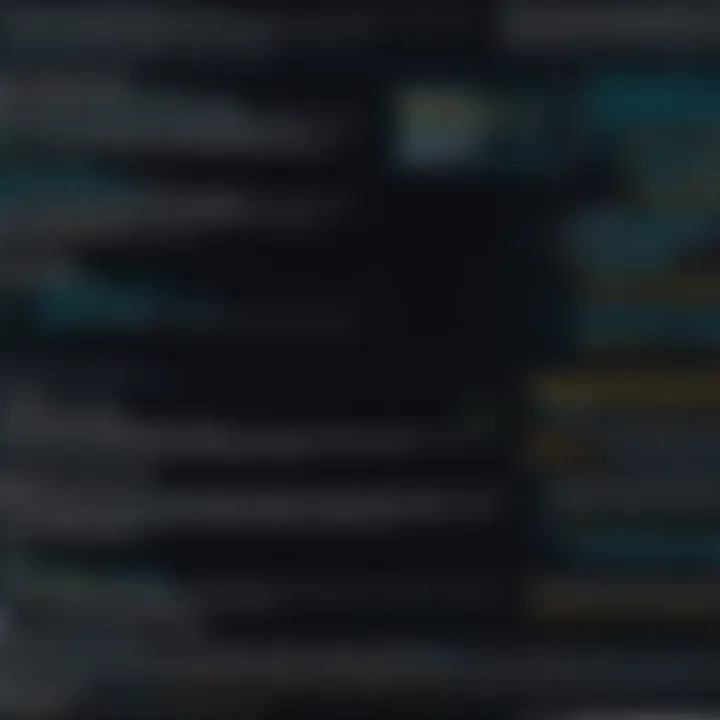
Intro
Text mining has carved a significant niche in the modern data analysis landscape, transforming the way organizations derive insights from unstructured data. In a world overflowing with information—from social media chatter to vast troves of documents and reports—extracting meaningful patterns has become both a challenge and a necessity. By employing text mining techniques, businesses and researchers can sift through this data deluge, honing in on valuable insights that drive decision-making and strategy.
The relevance of text mining spans across various sectors, offering unique applications that enhance customer engagement, streamline processes, and even inform healthcare decisions. Whether it's parsing customer feedback to tailor marketing strategies or identifying trends in medical literature, the potential applications are vast and varied. Here, we will explore not just the theoretical underpinnings but also practical implementations and the tools that support these endeavors.
As we delve deeper, readers—be they tech enthusiasts, IT professionals, or decision-makers in various industries—will gain a richer understanding of how text mining can be harnessed to achieve actionable intelligence. Let's now step into the realm of software that acts as the backbone of text mining processes.
Prelude to Text Mining
Text mining has become a cornerstone of modern data analysis. It involves extracting meaningful insights from large volumes of unstructured textual data, turning what can often seem like a chaotic mass of information into something actionable. In an age where data is produced at an unprecedented pace, the ability to make sense of this text-heavy data is not just beneficial; it's essential.
Defining text mining accurately sets the stage for understanding its implications and uses in various industries. This intricate process doesn’t merely involve the sifting through data but employs sophisticated analytics to discover patterns, trends, and insights that can guide decision-making. Whether it's parsing customer feedback or analyzing social media discussions, text mining serves as a critical tool that bridges the gap between raw data and actionable intelligence.
Defining Text Mining
Text mining, at its core, can be described as the process of uncovering hidden patterns in textual data. This includes various techniques from natural language processing, data mining, and machine learning. In simpler terms, think of it as having a very smart helper who reads and makes sense of all the documents, articles, and social media posts for you.
Consider, for example, the vast libraries of medical literature. Researchers face the daunting task of sifting through thousands of studies to find relevant data. Text mining tools can analyze these documents, highlighting connections and findings that might otherwise slip through the cracks. This definition underscores the practicality of text mining -- it’s not just a theoretical concept but a valuable service that enhances efficiency across many fields.
Importance of Text Mining in Modern Data Analysis
The importance of text mining cannot be overstated. First, it allows businesses to convert data into insights that are immediately applicable. Companies can gather and analyze customer sentiments from reviews, social media platforms, and surveys, enabling them to make informed strategic decisions.
Moreover, in the rapidly evolving tech landscape, where customer preferences and market trends shift like sand, text mining provides a way to predict these trends rather than merely react to them. Organizations must equip themselves with tools that enable them to stay a step ahead.
Here are several reasons why text mining is a game-changer for modern data analysis:
- Informed Decision-Making: Text mining helps in drawing insights that result in better business decisions.
- Efficiency Gains: Automating the process of data extraction saves time and reduces the potential for human error.
- Customization of Services: Understanding customer feedback allows for tailored marketing strategies.
- Research and Development: Facilitates quicker access to relevant research data, enhancing product development cycles.
"Text mining is not just about crunching numbers, but about producing knowledge from the vast sea of information."
If one wants to delve deeper into this fascinating subject, resources like Wikipedia offer foundational information, while platforms like Reddit provide community insights into real-world applications and advancements in text mining techniques.
In summary, the ability to extract distinct insights from unstructured text is a powerful capability that enhances the value of data analysis globally. Recognizing its importance sets the foundation for exploring its diverse applications across various fields.
Applications in Marketing
Exploring how text mining applies to marketing reveals just how integral this technology is in understanding consumer behavior, optimizing marketing strategies, and ultimately enhancing revenue. As companies navigate an increasingly complex landscape filled with data, the ability to extract insights from unstructured sources—like social media posts, customer reviews, and surveys—has become crucial. Not only does it help organizations stay relevant, but it also empowers them to anticipate market trends with greater accuracy.
The benefits of text mining in marketing can hardly be overstated:
- Enhanced Customer Understanding: Organizations can gauge customer preferences, sentiments, and pain points through mined text. This deep understanding allows for more personalized and effective marketing solutions.
- Efficient Resource Allocation: With insights derived from text mining, companies can better direct their marketing budgets and efforts where they'll yield the most benefit.
- Real-Time Market Insights: The speed with which data can be processed enables businesses to act quickly to shifts in consumer behavior and market demands.
Customer Sentiment Analysis
Diving into customer sentiment analysis, we see its pivotal role in interpreting the emotions behind customer feedback. It's more than just tallying stars on a review; it's about understanding the why behind those ratings. Companies can tap into various data sources, such as Twitter feeds, online reviews, or even call logs, and employ text mining techniques to assess consumer emotions and attitudes towards products or brands.
Utilizing sentiment analysis enables marketers to:
- Identify key trends in customer emotions, helping them refine product offerings based on actual feedback.
- Respond proactively to customer dissatisfaction, possibly turning a negative experience into a positive one.
- Monitor brand health over time, alerting them to potential PR issues or market shifts early on.
Targeted Advertising Strategies
Text mining can also significantly enhance targeted advertising strategies. By analyzing customer data and interactions across various platforms, marketers are able to tailor advertisements that resonate with specific audience segments. This prevents the often-dodgy approach of broad marketing campaigns from hitting the wrong notes.
Key advantages include:
- Personalized Content Delivery: Ads designed from mining data can appeal directly to individual preferences and interests.
- Increased Conversion Rates: By delivering the right message to the right audience at the right time, companies can see a marked increase in engagement and sales.
- Cost-Effective Advertising: With clearer insights, businesses can streamline their ad spend and focus on methods that yield better results.
Market Research Insights
Lastly, text mining serves as an extraordinary tool for extracting insights from extensive market research. It can assist companies in identifying emerging trends, consumer demands, and competitive landscape. By sifting through a mountain of textual data—including market reports and customer surveys—marketers can derive actionable insights that inform strategic decisions.
Some noteworthy impacts are:
- Richer Data Analysis: Unstructured data can be transformed into structured insights, offering a more comprehensive view of market conditions.
- Fostering Innovation: Understanding emerging trends allows companies to innovate effectively, staying one step ahead of competitors.
- Greater Decision-Making Confidence: By relying on hard data rather than gut feeling or anecdotal evidence, marketing leaders can forge ahead with strategies they believe in.
"In the era of big data, the companies that can best harness the power of unstructured information will thrive."
In essence, the adoption of text mining within marketing is a game-changer, enhancing how organizations understand their customers and respond to market dynamics. As they look into the horizon, it is clear that implementing text mining strategies will be an essential element in developing a competitive edge.
Text Mining in Healthcare
Text mining within the healthcare sector is a vibrant and growing field. As the healthcare industry generates and collects vast amounts of data, the need for a methodical approach to analyze this information becomes essential. This analysis helps in decision-making, improving patient care, and enhancing operational efficiency. The implications of text mining are not just theoretical; they manifest in real-world improvements that positively affect both healthcare providers and patients alike.
Patient Data Processing
In healthcare, patient data is not just numbers; it's a rich source of information waiting to be decoded. Text mining plays a pivotal role in processing unstructured data, like clinical notes, discharge summaries, and electronic health records. By applying natural language processing techniques, healthcare analysts can extract relevant information regarding patient histories, treatment outcomes, and potential risks.
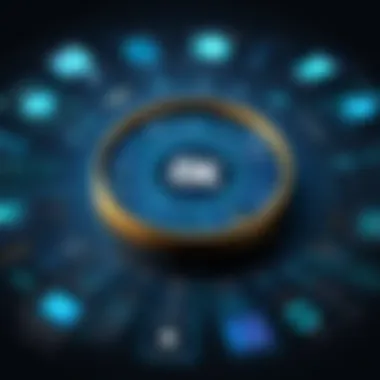
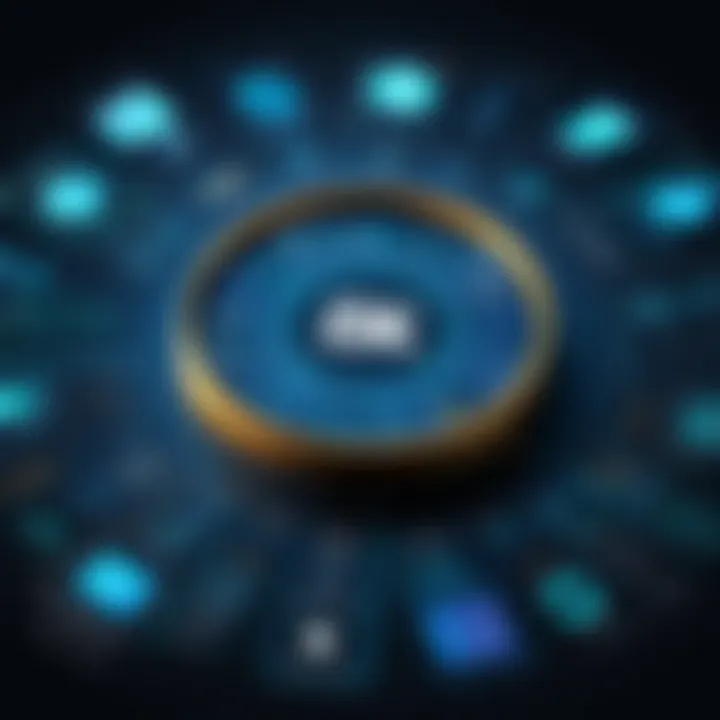
Imagine a situation where a doctor accesses a patient's electronic file and is confronted with pages of notes. Instead of manually sifting through, text mining tools can summarize key points, highlight medications, and note any allergies or conditions. This results in quicker, data-driven decision-making.
Furthermore, by automating the extraction of data from diverse sources, organizations can significantly reduce the likelihood of human error and improve patient safety. More accurately processed data fosters enhanced continuity of care, leading to better health outcomes.
Medical Literature Review
Consider the amount of research published in medical literature each day. For a healthcare professional, staying updated can feel like drinking from a fire hose. Text mining helps in categorizing and summarizing vast quantities of research articles.
Using text mining technologies, researchers can analyze trends across studies, evaluate treatment effectiveness, and identify best practices in patient care. This not only saves crucial time but also provides insight into treatment modalities that may be more effective for certain conditions. Automated review processes can highlight connections and gaps in research that might otherwise be overlooked, fostering collaboration across various specialties.
Text mining in literature reviews can significantly expedite the synthesis of information, turning a mountain of data into actionable insights.
Predictive Analytics in Patient Outcomes
The predictive aspect of text mining is where the real magic happens in healthcare. By analyzing historical patient data, healthcare providers can predict potential outcomes and identify at-risk populations. These insights are invaluable when it comes to planning interventions before problems escalate.
For instance, by combining patient demographics with historical data on similar cases, hospitals can develop predictive models to foresee potential readmissions. This enables tailored follow-up care, offering a higher likelihood of successful outcomes. Further, predictive analytics can play a vital role in resource allocation, ensuring that healthcare facilities can meet patient needs without strain.
In summary, the use of text mining in healthcare not only reduces time and labor but also turns unstructured data into structured insights that lead to informed decisions and improved patient care.
Through thorough analysis of patient data processing, literature reviews, and predictive analytics, we recognize that harnessing these methods is crucial for modern healthcare practices. It ensures that healthcare professionals are not left guessing but are enabled with data to guide their decisions.
Financial Services Applications
Financial services have always strived to optimize operations and enhance customer experiences. In the age of information, text mining emerges as a powerful ally. This technology allows financial institutions to sift through mountains of unstructured data, from transaction records to regulatory documents, extracting actionable intelligence. The implications of harnessing text mining in this sector are far-reaching. It can lead to significant cost savings, improved decision-making, and enhanced compliance with regulations. As such, understanding the applications of text mining in finance is crucial for anyone looking to keep pace with industry trends.
Fraud Detection Models
Fraud in the financial sector can have severe consequences, not just for the institution, but also for customers. To combat this, companies increasingly rely on fraud detection models powered by text mining. These models analyze patterns and anomalies in transaction data, alongside textual data like customer communications. For example, a sudden change in transaction style or volume can trigger an alert, enabling swift investigation. Notably, text mining incorporates natural language processing to scrutinize customer interactions, flagging linguistic cues that may indicate deceit.
Some commonly used methods include:
- Machine Learning Algorithms: Using historical data, the models can learn what constitutes normal behavior.
- Rule-Based Systems: These systems apply predefined rules to identify suspicious activity swiftly.
- Anomaly Detection: Detecting outliers helps identify unusual patterns that warrant further investigation.
The combination of these techniques enriches the model’s accuracy, ensuring timely responses to fraudulent activities, thus protecting both the institution and its clientele.
Investment Trend Analysis
Investors and analysts are always on the lookout for the next big opportunity. Text mining provides them with the tools to analyze market announcements, news articles, analyst reports, and even social media chatter. By identifying patterns in textual data, firms can gauge market sentiment and predict investment trends that can influence stock prices and market directions.
The essential facets of investment trend analysis aided by text mining include:
- Sentiment Analysis: Assessing public and expert opinion helps investors make informed choices.
- Topic Modeling: Clustering similar topics allows for understanding overarching market themes.
- Event Modeling: Analyzing the impact of specific events on market conditions enables more accurate predictions.
For instance, if there’s a surge in positive sentiments surrounding a tech company, it could signal potential growth, guiding analysts to recommend stock purchases.
Risk Assessment Tools
In finance, risk management is paramount. Text mining enhances risk assessment by analyzing regulatory compliance documents, historical data, and current market sentiments. It helps categorize risk types and understand their potential impact on the organization. The larger the dataset, the more accurate the risk modeling.
Essential components include:
- Quantitative Risk Metrics: Leveraging data to calculate potential losses based on historical trends.
- Qualitative Analysis: Understanding the context surrounding risks through stakeholder communications.
- Continuous Monitoring: Ongoing analysis allows for real-time risk assessment rather than relying solely on past data.
Using text mining to develop risk profiles enables companies to tailor their risk management approaches, ensuring they can respond more effectively to emerging threats.
In summary, the financial sector's embrace of text mining underscores its pivotal role in enhancing fraud detection, discerning investment trends, and refining risk assessment approaches. This technology not only facilitates better decision-making but also arms financial institutions against evolving challenges.
Text Mining in Social Media
The landscape of social media is a bustling hub where opinions, trends, and sentiments fly about like confetti in a parade. In this environment, text mining proves to be an invaluable resource, sifting through the noise to extract meaningful insights. Social media platforms such as Twitter, Facebook, and Reddit allow users to share thoughts instantly, creating a mountain of text data ripe for analysis. This section examines how text mining is deployed to identify trends, manage crises effectively, and monitor brand reputation in an age where public perception can shift faster than a New York minute.
Trend Identification and Analysis
Identifying trends on social media isn't just about guessing what’s popular; it's a sophisticated process enhanced by text mining techniques. By analyzing hashtags, keywords, and user interactions, businesses can detect emerging patterns. This could be as simple as noticing an uptick in conversations around plant-based diets or as complex as analyzing sentiment shifts regarding political issues.
For instance, social listening tools utilize natural language processing (NLP) to scan and comprehend vast amounts of unstructured text. The insights gleaned can be leveraged for various purposes, including product launches or marketing campaigns. More importantly, understanding trends allows companies to stay ahead of the curve, ensuring they remain relevant and responsive to audience preferences.
- Benefits of Trend Analysis:
- Early identification of market shifts
- Tailored marketing strategies based on consumer behavior
- Enhanced engagement with target audiences
Crisis Communication Management
In the realm of social media, a crisis can escalate faster than a speeding bullet. Text mining plays a pivotal role in crisis communication, aiding organizations in identifying potential issues before they blow up. By monitoring sentiment and key phrases associated with their brand, companies can detect early signs of distress.
Consider a scenario where a product fails or a service goes awry. Immediate feedback from social media can scrutinize customer sentiments in real-time, allowing companies to react quickly. An established text mining framework enables organizations to categorize and prioritize these mentions, streamlining response efforts.
For example, if sentiment analysis reveals a negative shift in feedback during a product issue, a well-articulated response can mitigate rising tides of backlash.
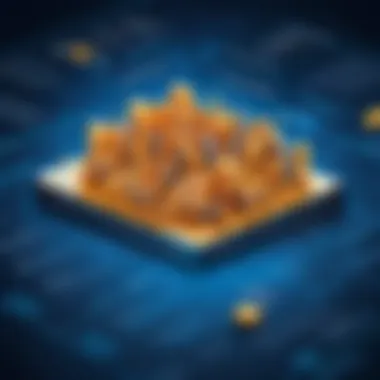
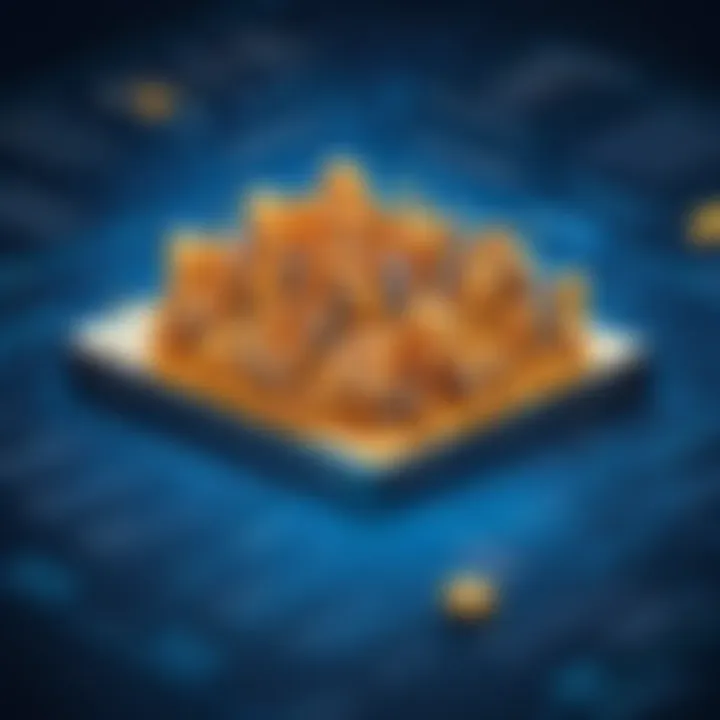
"Effective crisis management hinges on how swiftly organizations can collect and analyze feedback to transform challenges into actionable strategies."
Brand Reputation Monitoring
In an era where a single tweet can alter a brand's reputation overnight, monitoring this reputation has never been more crucial. Text mining aids organizations in assessing public perception by continuously scanning social media for mentions, reviews, and discussions related to their brand.
By employing sentiment analysis algorithms, businesses can differentiate between positive and negative mentions, giving them insight into their audience's feelings. The ability to react promptly not only helps in damage control but fosters a positive relationship between the brand and its audience.
- Key Techniques for Monitoring Brand Reputation:
- Real-time sentiment analysis
- Keyword tracking to catch mentions early
- Comparative analysis with competitors to gauge market position
This proactive stance not only promotes transparency but can also be translated into actionable changes that enhance customer satisfaction and loyalty.
Insights from Customer Feedback
In the digital age, understanding consumer sentiment and preferences is paramount. The insights derived from customer feedback not only inform business strategies but also shape product offerings and marketing approaches. Text mining plays a critical role in this process, helping organizations to sift through vast amounts of unstructured data. The ability to analyze reviews, social media posts, and surveys enables companies to grasp what their customers are genuinely feeling and thinking.
Understanding User Experience
Diving deeper into the realm of user experience, text mining helps to translate qualitative feedback into quantifiable data. Every review, comment, or post on social platforms carries within it a wealth of information that can indicate customer satisfaction or dissatisfaction. By applying techniques such as sentiment analysis, businesses can categorize feedback into positive, negative, or neutral sentiments. This categorization is not merely about counting likes or dislikes but involves understanding the why and how behind those sentiments.
For example, a simple comment like "The battery life is fantastic!" speaks volumes. Text mining can pull in this data and highlight it as a significant quality differentiator. On the other hand, a customer saying, "I wish this product had more features" signals an area for improvement. Recognizing these subtle indicators can empower teams to enhance user experience significantly.
Improving Product Development Cycles
The iterative nature of product development requires continuous feedback loops to meet consumer needs. Text mining allows businesses to incorporate customer opinions into their development processes seamlessly. It aids in recognizing trends over time—what worked last season may not suffice today. Through analyzing historical feedback, organizations can unearthed valuable insights to guide their product roadmaps.
Such methods can lead to better decision-making concerning which features to prioritize or eliminate. A notable increase in feedback about a feature also provides a testament to its importance, encouraging development teams to focus their efforts.
In practice, suppose a tech company is launching a new smartphone. By leveraging text mining to analyze feedback from beta testers, they might discover a recurring theme: users find the camera interface confusing. Rather than guesswork, the company can act on concrete data to enhance that aspect before release.
In summary, exploring insights from customer feedback through text mining offers a dual advantage. It not only informs organizations about their current standing with consumers but also equips them with necessary knowledge to foster innovation and improvement. This iterative refinement ultimately leads to higher customer satisfaction and loyalty.
Effective use of customer feedback can lead to smarter products and superior user experiences, making text mining an invaluable asset for businesses today.
Text Mining in Academia
Text mining in academia has increasingly become a cornerstone for various research initiatives across disciplines. This technique employs rich algorithms and software tools to extract pertinent information from vast text datasets, turning seemingly chaotic information into structured forms that researchers can easily navigate and utilize. As academics face the growing challenge of sifting through mountains of literature, text mining provides an efficient solution, allowing scholars to focus on interpretation and analysis rather than merely data collection.
The benefits of incorporating text mining methodologies into academic research are profound. Firstly, researchers can identify emerging trends and patterns that may not be immediately clear through traditional analyses. This can be particularly advantageous in fast-evolving fields such as social sciences, medicine, and technology, where timely insights are vital. Furthermore, the ability to harness insights from a wide range of literature, from journals to conference proceedings, enhances both quantitative and qualitative research, thereby enriching the overall scholarly conversation.
In addition to trend analysis, text mining further streamlines the academic writing process. By efficiently organizing vast bodies of knowledge, text mining allows scholars to summarize and categorize existing literature, facilitating easier citation and reference. This approach not only improves writing quality but also ensures that important works are not inadvertently overlooked.
Analyzing Research Trends
One of the primary functions of text mining in academia is the ability to analyze research trends effectively. By employing methods like topic modeling and sentiment analysis, researchers can pinpoint key areas of interest or concern within a field. For instance:
- Topic Modeling: Algorithms like Latent Dirichlet Allocation (LDA) can categorize large sets of documents into topics. This assists researchers in understanding what subjects are gaining traction and which might be waning.
- Citation Analysis: By examining citation patterns, scholars can identify influential papers, gauge the impact of research over time, and observe how specific ideas or theories evolve.
- Keyword Extraction: Automatically identifying prominent keywords in research publications helps in rapidly assessing current focuses and gaps within a discipline.
Utilizing these methods not only saves significant amounts of time but also provides a clearer view of the academic landscape, enabling researchers to align their efforts with ongoing discussions.
Text Mining for Academic Writing
Text mining also plays a crucial role in enhancing the academic writing process itself. By integrating various text mining tools, scholars can improve their writing in several impactful ways:
- Literature Review Automation: Text mining makes it simpler to conduct comprehensive literature reviews. This is achieved by quickly gathering and summarizing relevant research findings, which saves hours of manual searching and reading.
- Plagiarism Detection: Using these tools, researchers can ensure that their work is original by cross-checking their text against extensive databases to flag potential similarities with other works.
- Improved Structure and Cohesion: Using data-driven insights, scholars can structure their writing to align better with successful frameworks observed in highly cited papers. This focus on structure can enhance their visibility and engagement in academic circles.
By leveraging text mining, academics can tap into a wealth of information that improves both the depth and breadth of their work, ultimately fostering innovation and collaboration in their respective fields.
In sum, text mining in academia not only streamlines the process of research and writing but also enhances the quality and relevance of academic output. As the amount of information continues to grow exponentially, adopting these techniques is no longer optional; rather, it’s a necessity for maintaining relevance in scholarly conversations.
Legal Applications of Text Mining
In the legal field, the sheer volume of documents and data can be overwhelming. This is where text mining comes into play, offering significant advantages for legal practitioners. By extracting relevant information from unstructured data, text mining aids in greater efficiency and accuracy in legal processes.
Leveraging this technology enables law firms to enhance their due diligence processes, streamline extensive legal research and optimize case management. This not only saves valuable time but also helps in making informed decisions based on rich insights drawn from prior case laws, contracts, and legal documents.
Contract Analysis and Review
Contract analysis is one area where text mining can greatly simplify work for legal professionals. With contracts often containing dense legal language and technical terms, mining tools can flag critical clauses and potential risks, ensuring nothing slips through the cracks. This can also include:
- Identifying key terms and conditions which could influence negotiations.
- Highlighting any regulatory compliance issues that may arise from specific contract language.
- Streamlining contract reviews by summarizing lengthy documents into concise points.
These capabilities address some of the biggest pain points in contract management. Traditional methods rely heavily on manual review, which is labor-intensive and prone to human error. For instance, if a lawyer misreads a critical clause in a contract, it could cost their client significantly in legal disputes or financial penalties.
By integrating text mining solutions, law firms can transform how they assess and manage contracts. Once thought impractical, the technology now allows lawyers to focus on higher-level strategic thinking, rather than poring over minutiae in endless paperwork.
Case Law Research
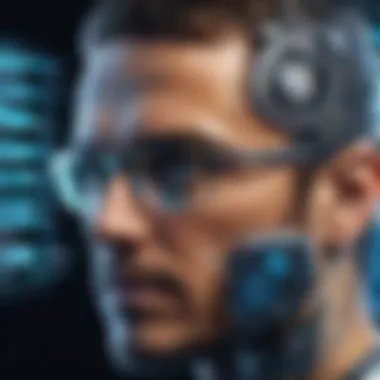
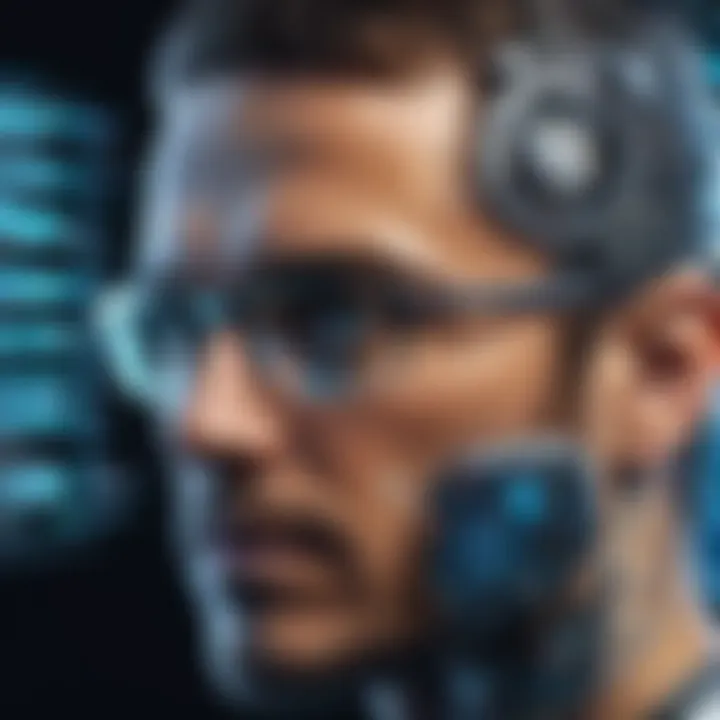
In the realm of case law research, text mining stands as a game changer. Historically, legal professionals spent countless hours scouring through archives and databases to find relevant precedents. Text mining algorithms can sift through large quantities of legal documents and case law databases, returning relevant results almost instantaneously.
Some significant advantages of using text mining in case law research include:
- Efficiency: Reduces the time spent on routine searches, allowing lawyers to dedicate more time to preparing their arguments.
- Accuracy: Incorporates data-driven insights to ensure that no important case law is overlooked.
- Trend Analysis: Enables law firms to identify relevant ongoing trends in litigation and develop strategies based on empirical data.
In summary, both contract analysis and case law research showcase how the legal industry can benefit immensely from text mining. By embracing this technology, legal professionals can stay ahead of the curve, ensuring their clients receive effective and timely counsel.
"Text mining is no longer a luxury for legal professionals; it’s a necessity to keep pace with the rapidly evolving legal landscape."
Overall, the implementation of text mining in legal applications not only enhances productivity but also fosters better compliance with regulations and facilitates risk management. As this technology continues to evolve, it offers exciting prospects for transforming legal workflows and improving outcomes.
Challenges in Text Mining Applications
In the realm of text mining, navigating through the complexities can feel like traversing a dense forest. Much like the thicket many users encounter while seeking clarity within unstructured data, several challenges come to the forefront. Understanding these obstacles isn't merely an exercise in criticism; it’s vital for enhancing the efficacy of text mining and, by extension, achieving reliable insights that can inform business strategies or academic research. Ignoring these challenges may lead to misguided conclusions, skewed results, or even unethical practices, underscoring the necessity of addressing them head-on.
Data Quality Issues
At the heart of any successful text mining process lies data quality. If the data you input is riddled with errors, the end results are likely to be less than stellar. Poor quality data can stem from various sources, including typos, slang, and inconsistent terminology. For instance, when analyzing customer feedback, if a vast proportion of responses contain misspellings or unusual phrases, it can throw a wrench into the analysis.
Moreover, noise can infiltrate datasets, which often complicates the analytical process. This noise can refer to irrelevant content, such as advertisements interspersed within user comments, or even data that’s simply outdated. Notably, a study garnered from healthcare forums illustrated how varying definitions of medical conditions complicated the analysis of patient experiences, ultimately diluting the medical insights that could have been gleaned.
To mitigate these issues, organizations might undertake strategies such as:
- Data cleansing: Identifying and correcting inaccuracies.
- Standardization: Implementing common structures and formats to facilitate easier analysis.
- Data augmentation: Enriching datasets with external data to improve coverage and richness.
By confronting data quality head-on, businesses can ensure they find their footing in this analytical landscape, enhancing the value derived from text mining initiatives.
Ethical Considerations
Ethics in text mining isn’t just an afterthought; it’s crucial to maintain trust and integrity in data practices. The way data is collected and analyzed can raise numerous ethical questions, particularly concerning privacy concerns. For instance, let’s say a company decides to mine social media posts for sentiment analysis. While customers willingly share their thoughts, they may not expect those thoughts to be analyzed for commercial purposes. Misunderstanding this can lead to a severe distrust among users, perhaps even resulting in backlash.
Additionally, there are biases inherent to data that can adversely affect outcomes. Algorithms might learn to 'favor' certain demographics due to historical data imbalances. If one examines reviews predominantly from a particular socioeconomic class without accounting for the diversity within the larger population, the outcome may not reflect broader sentiments.
To address these ethical dilemmas, organizations should consider:
- Transparent communication: Clearly informing users about how their data will be used.
- Bias identification and prevention: Actively analyzing datasets for potential biases before deploying algorithms.
- Regular audits: Continually assessing data practices to ensure compliance with ethical standards.
Addressing these ethical considerations is not merely a regulatory necessity, but can also result in remarkable customer loyalty and brand strength.
In summation, while text mining holds the promise of delivering remarkable insights, acknowledging challenges related to data quality and ethics serves as foundation for sustainable and responsible application. With careful navigation, professionals can harness the full potential of text mining while maintaining integrity and accuracy.
Future Trends in Text Mining
The realm of text mining is on the cusp of transformative trajectories, reflecting both technological advancements and the evolving needs of industries. As data continues to proliferate, understanding the future trends in text mining is critical for both software developers and businesses. This section dives into two pivotal areas: the integration of machine learning and artificial intelligence and the development of enhanced predictive models.
Integrating Machine Learning and AI
At the heart of advancing text mining capabilities is the incorporation of machine learning and artificial intelligence. These technologies are revolutionizing how data is processed and interpreted. In particular, they enable systems to learn from vast amounts of unstructured data, identifying patterns that were previously invisible to human analysts.
- Automated Classification: Algorithms can be trained to automatically categorize text into predefined groups, vastly improving efficiency. For businesses, this means faster processing times for customer feedback, support tickets, or social media mentions.
- Natural Language Processing: By leveraging AI, text mining tools can improve their understanding of context, sentiment, and nuance in human language. This development is crucial for applications in customer sentiment analysis and content creation.
- Real-time Analytics: With AI at the helm, text mining can deliver insights in real time, fostering quicker decision-making and allowing businesses to respond to emerging trends or crises without delay.
Incorporating machine learning isn't just a trend. It's a necessity for maintaining a competitive edge in data-driven markets. Companies fail to harness AI effectively risk falling behind their more agile peers.
Enhanced Predictive Models
The next frontier in text mining is the development of enhanced predictive models that take into account more sophisticated algorithms and a broader range of data inputs. Here’s why these models matter:
- Complex Data Relationships: Enhanced models can analyze intricate relationships within the data that simpler models might overlook, providing deeper insights and more accurate forecasts.
- Adaptive Learning: These predictions adapt based on historical data, meaning they improve over time. The more data they process, the better they become at anticipating future trends or behaviors.
- Interdisciplinary Approaches: By integrating insights from various fields—like psychology, economics, and sociology—models become richer and more applicable across sectors. This sort of cross-pollination of ideas can lead to groundbreaking innovations in how businesses interact with their customers.
"The integration of advanced predictive modeling techniques into text mining signifies a shift from reactive strategies to proactive engagement across industries."
As we move forward, companies that proactively invest in these future trends will find themselves reaping the rewards of more efficient data processing, improved customer insights, and greater innovation.
Understanding the underpinning of these shifts can dramatically enhance the way organizations not only gather data but also how they utilize that information to drive strategic decision-making.
Ending
The conclusion of this article serves as a crucial summation of the multifaceted role of text mining across different industries. It ties together the various applications discussed and highlights the overarching significance of harnessing unstructured data in a way that brings tangible value to organizations. One cannot underestimate the benefits that text mining offers; whether it's enhancing customer engagement in marketing or predicting patient outcomes in healthcare, the insights gleaned can influence strategic decisions profoundly.
Summarizing Key Insights
To recap, the key insights from this article showcase the versatility of text mining. It functions as a bridge connecting raw data with actionable intelligence. Here are several important points:
- Diverse Applications: Text mining is employed across various sectors—from marketing to finance and healthcare.
- Data-Driven Decision Making: Organizations leverage text mining to make informed choices, minimizing risks and capitalizing on opportunities.
- Machine Learning Integration: The coupling of text mining with machine learning enhances predictive capacities, making it an invaluable asset for future trends.
- User Experience Enhancement: By analyzing customer feedback, businesses can refine their products and services, leading to higher satisfaction rates.
Understanding these insights positions practitioners to capitalize on the advantages text mining offers.
Implications for Practitioners
For practitioners, the implications of deploying text mining technologies are profound. Here are several key takeaways:
- Strategic Implementation: Practitioners need to approach text mining as a strategic asset, embedding it within their organizational framework to optimize productivity.
- Continuous Learning: The landscape of text mining technology is constantly evolving. Staying abreast of new developments is crucial for maintaining a competitive edge.
- Ethical Considerations: As they harness insights from user data, practitioners must remain vigilant about ethical practices, ensuring that data privacy stays intact.
- Adaptability: Medium to large enterprises should remain adaptable in their text mining strategies, adjusting to feedback and market shifts to ensure relevance.
By summarizing these crucial points in the conclusion, the article encapsulates the essence of text mining as not just a technical endeavor, but as a fundamental driver of innovation and efficiency in today’s data-centric world.