Behavioral Cohort Analysis for User Insights
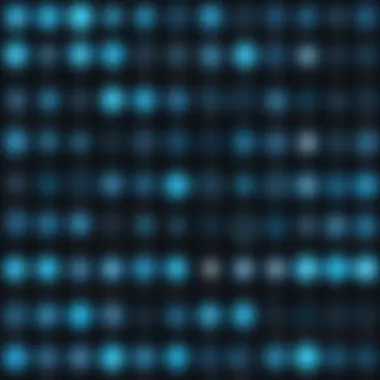
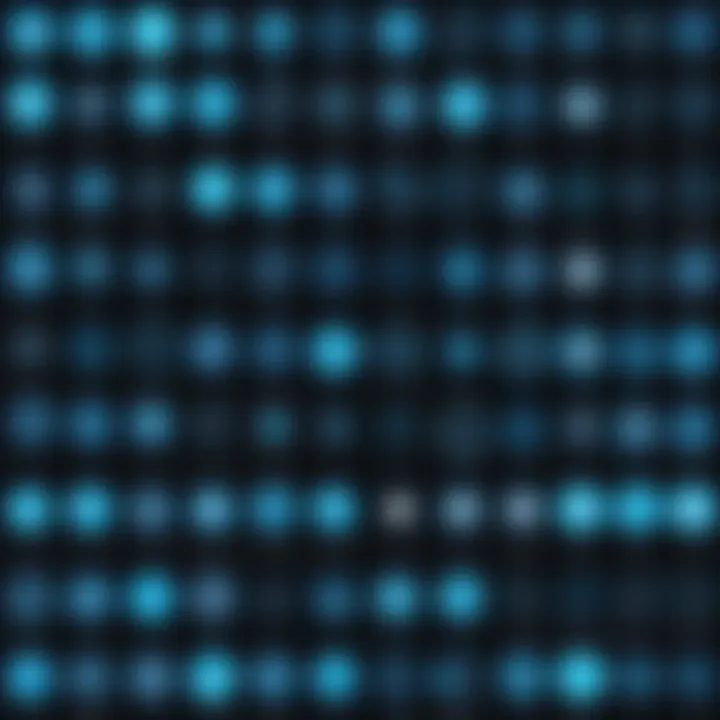
Intro
In today’s data-driven environment, understanding user behavior is vital for software developers and business leaders alike. User engagement can significantly influence how a product or service is perceived and ultimately its success in the marketplace. That's where behavioral cohort analysis comes into play. This methodology goes beyond surface-level metrics and dives deep into user interactions, providing valuable insights to inform better decisions.
But what exactly is behavioral cohort analysis, and why should software professionals consider it a key tool in their arsenal? It’s all about segmenting users into distinct groups based on their shared behaviors or interactions with the software. This enables targeted analysis that can lead to actionable insights. By identifying trends and patterns distinct to each cohort, organizations can tailor their strategies to maximize engagement and retention.
Why Cohort Analysis Matters
Cohort analysis is not just another buzzword in the tech landscape. It holds serious value for both small startups and large enterprises. The insights gained can shape marketing strategies, influence product development, and enhance user experience. Understanding the uniqueness of each user segment helps in creating personalized experiences. This is crucial in an age where consumers expect tailored solutions that resonate with their specific needs.
Let's break down how behavioral cohort analysis works, examine its key benefits, and see how it can elevate comprehension of user dynamics.
Prelims to Behavioral Cohort Analysis
Understanding user behavior is no longer just a luxury for modern businesses; it’s a necessity. Behavioral cohort analysis emerges as a crucial strategy in this context, offering a nuanced way to explore how different groups of users interact over time. Gone are the days when general statistics reigned supreme—specificity is the name of the game here.
By defining and examining behavioral cohorts, organizations can tap into a deeper pool of insights. Think of it as looking through a prism instead of just a window. Each cohort represents a unique subset of users, marked by shared characteristics or behaviors. This form of analysis doesn't merely scratch the surface; it digs deeper, revealing layers of trends and patterns that can significantly influence business strategies.
Defining Behavioral Cohorts
At its core, a behavioral cohort consists of users grouped by shared experiences or interactions within a certain timeframe. These can include those who signed up in a particular month, people who made repeated purchases, or users engaging actively with specific features.
Understanding what defines these cohorts is vital. For example, let's say you run a project management tool. You might want to segment users into cohorts based on whether they frequently utilize collaboration tools or project timelines.
This segmentation allows for insightful comparisons. If one group consistently demonstrates high engagement, organizations can analyze what makes that cohort tick, leading to targeted enhancements in user experience or product features.
Importance in User Engagement Strategies
In the ever-competitive landscape of software solutions, user engagement becomes a decisive determinant of success. Behavioral cohort analysis not only helps track engagement but also enables tailoring strategies that resonate with specific user behaviors. A well-structured engagement plan can be the difference between satisfied users and those who feel left in the dust.
Moreover, the findings from this analysis can illuminate potential pitfalls. For instance, if a certain cohort shows a decline in engagement, it’s a warning sign that something may need fixing. This predictive capability equips stakeholders with the tools they need to address problems proactively.
"Cohorts tell the story of user journeys. Without them, you’re just wandering in the dark, hoping to stumble upon what works."
It's also worth noting how the insights gleaned can bolster marketing strategies. By understanding behavioral patterns, campaigns can be streamlined to address specific segments, leading to higher conversion rates and more personalized marketing efforts. In a world where consumers are bombarded with generic messages, personalization can pave the way for stronger connections.
In summary, the introduction of behavioral cohort analysis marks a paradigm shift in understanding user engagement. This approach brings clarity and specificity to how different groups of users interact, which is crucial for designing effective strategies that cater to diverse user needs.
The Methodology of Behavioral Cohort Analysis
Understanding the methodologies behind behavioral cohort analysis is essential for any organization aiming to unpack the complexities of user behavior. This process involves a structured approach that helps in dissecting users into various cohorts based on their behaviors and interactions with the product. It ultimately benefits the organization through improved user engagement and strategic targeting.
Data Collection Techniques
Data collection forms the backbone of effective behavioral cohort analysis. Various techniques can be employed, each with its own strengths and weaknesses. Here’s a glimpse into some effective data collection methods:
- Surveys and Questionnaires: One of the simplest ways to gather insights directly from users. Depending on how they're crafted, surveys can reveal a lot about user preferences and satisfaction levels.
- Web Analytics Tools: Tools like Google Analytics help track user behavior on websites, allowing for a granular view of user interactions, including page views, click rates, and bounce rates.
- Event Tracking: Using tools like Mixpanel or Amplitude allows businesses to track specific user actions in real-time. This method highlights patterns in engagement across different touchpoints.
- User Interviews and Focus Groups: Engaging directly with a sample of users provides qualitative insights that numbers alone can't illuminate. Here, casual conversation can yield qualitative data that uncovers motivations and obstacles to use.
Implementing multiple data collection techniques creates a comprehensive view of user behavior, bringing valuable insights into the analysis.
Segmentation Approaches
Once the data is collected, the next step is segmentation. Not every user behaves in the same way, and recognizing these differences is crucial to tailoring strategies. Various approaches to segmentation can include:
- Demographic Segmentation: Dividing users into groups based on age, gender, location, or income level. It can be a useful first step in understanding general trends, although it often overlooks behavioral nuances.
- Behavioral Segmentation: This goes beyond demographics and involves categorizing users based on their interactions, such as purchase history or engagement patterns. It often provides deeper insights into what users really want.
- Psychographic Segmentation: This method incorporates users' values, interests, and lifestyles. It requires more subjective data but can yield unique insights that demographic data might miss.
- Temporal Segmentation: Recognizing how user behavior changes over time and in different contexts. For example, seasonality can significantly affect engagements and purchases.
Each segmentation approach opens unique avenues for analysis, enabling organizations to craft personalized experiences that resonate with distinct user groups.
Analytical Tools and Technologies
To make the most of behavioral cohort analysis, leveraging the right tools and technologies is critical. Here are a few to consider:
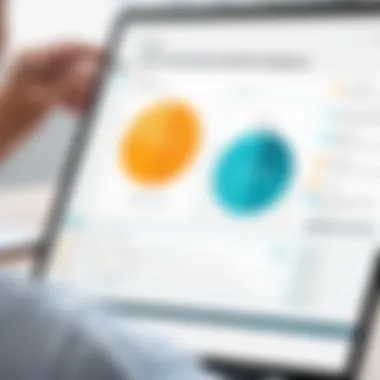
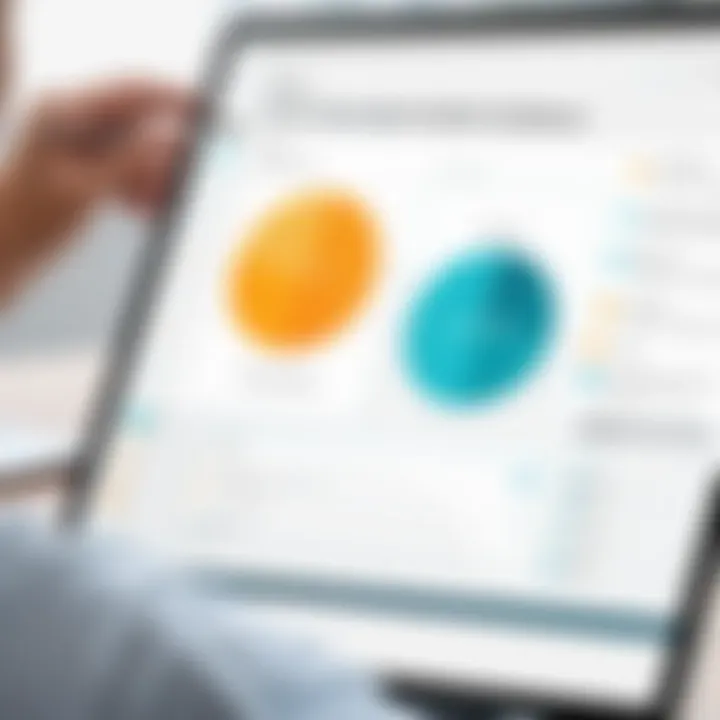
- Customer Relationship Management (CRM) Systems: Platforms like Salesforce or HubSpot can centralize user data and allow for seamless tracking of interactions across different touchpoints.
- Business Intelligence Tools: Solutions such as Tableau or Power BI can assist in visualizing complex data sets, providing clear insights that aid in strategic decision-making.
- Machine Learning Algorithms: These can help predict future behaviors based on past data, automating a large part of the analysis and making it more efficient.
- A/B Testing Tools: Tools like Optimizely or VWO enable organizations to test different strategies and understand what resonates best with users, ultimately refining engagement tactics.
Combining these technologies not only streamlines the analytical process, but also enhances accuracy and insight depth, leading to more informed user engagement strategies.
"Behavioral cohort analysis requires a multi-faceted approach to truly understand the users—combining data collection, segmentation, and advanced analytics provides the clarity needed for impactful decision-making."
By grasping the methodologies outlined above, organizations can develop a robust foundation for understanding their users on a deeper level, significantly enhancing their strategic capabilities in software evaluation.
Applications of Behavioral Cohort Analysis in Software Review
Behavioral cohort analysis plays a pivotal role in understanding user interactions with software products. It helps categorize users into specific groups based on their behaviors rather than just demographic factors. This method enables businesses to make more informed decisions that ultimately enhance user experience and retention. The benefits are manifold, particularly in the realm of software evaluation. Businesses can tailor their approaches uniquely, ensuring they meet the demands of their diverse user base.
Enhancing User Experience Design
In the context of user experience design, applying behavioral cohort analysis allows designers to pinpoint what resonates with different user segments. By observing how groups with similar behaviors engage with a software application, product teams can refine elements that might be underperforming. For instance, a software tool might have a feature that appeals to power users but confuses novice users. Analyzing the behavior of these cohorts provides insight into necessary design modifications that enhance clarity and usability.
When developing user-centered designs, consider the following:
- Feedback Loops: Establish feedback loops with users. Understanding their pain points and desires via cohort-specific surveys can direct design choices that contribute to overall satisfaction.
- User Journey Mapping: Visualizing different user journeys can lead to the identification of moments where engagement drops. Adjustments can then be made to improve these critical areas, resulting in a smoother user experience.
Cohort analysis transforms the design process, enabling teams to create experiences that are not just attractive but functional for varied users.
Tailoring Marketing Strategies
When delving into marketing strategies, behavioral cohort analysis serves as a backbone for targeted campaigns. By segmenting users based on behaviors, marketers can craft messages that truly resonate with specific audiences. For instance, if a certain cohort is predominantly using a specific feature of a tool, promotional efforts can highlight this functionality in marketing materials directed at them.
Effective stratagies for applying this analysis include:
- Personalized Campaigns: Deploy personalized email marketing campaigns based on user activity. If users exhibit certain behavior patterns, suggesting related features or up-sells can accelerate engagement.
- Predictive Analysis: By analyzing past behaviors, businesses can forecast future actions, allowing them to adjust their promotional efforts accordingly.
Enhancing marketing strategies using cohort analysis not only increases conversion rates but also fosters long-term customer loyalty.
Improving Product Development Processes
Cohort analysis enriches product development by providing data-driven insights that guide feature enhancements and new capabilities. By understanding the distinct behavioral patterns of varied user segments, teams can decide which features to prioritize. This means resources are spent on changes that actually matter to users instead of making assumptions.
Practical implementations include:
- Iterative Development Based on Feedback: Regularly collect and analyze user behavior data to inform product iterations. It prompts teams to focus on enhancements that address real user needs.
- Beta Testing with Specific Cohorts: When launching new features, testing with user segments that will most likely benefit from these changes ensures accuracy in feedback and generates valuable insights.
Understanding User Segmentation
User segmentation forms the backbone of effective behavioral cohort analysis. The way individuals engage with software is not a one-size-fits-all scenario. Instead, each user presents a unique set of behaviors and preferences that, when understood thoroughly, can lead to better product alignment and marketing efficiency.
At its core, segmentation allows software professionals to categorize users based on specific characteristics or behaviors. This method can significantly enhance the decision-making process when it comes to designing, marketing, or modifying a software product. The ultimate goal is to create experiences that are not only user-friendly but also tailored to meet the distinct needs of different segments.
Demographics-Based Cohorts
Demographics can indicate a whole lot about user behavior. Age, gender, location, and income are just a few of the many demographic factors that can affect how users interact with software. For instance, a mobile app that's popular among millennials in urban areas might not resonate with older generations living in rural settings. This demographic insight helps developers make informed choices about features, marketing languages, and even customer support approaches.
- Benefits:
- Targeted Marketing: When knowing user demographics, teams can tailor marketing efforts to resonate with specific segments, thereby increasing conversion rates.
- Feature Customization: By analyzing cohesive demographic groups, products can be tailored to suit these specific users.
- Resource Allocation: Understanding who the users are allows companies to allocate resources where they will have the most impact.
Behavioral Traits and Preferences
Diving into behavioral traits offers another layer of understanding. Two users may fall within the same demographic group yet have completely different interactions with the product based on their behavioral tendencies. Are they heavy users or casual browsers? Do they engage with features more often than others? Such insight can inform future developments and marketing strategies.
- Key Considerations:
- User Journey Mapping: Analyze how different users navigate through your software. This can help in optimizing their journey.
- Engagement Triggers: Identify what prompts users to engage more deeply with the product, whether it’s notifications, feature updates, or personalized offers.
- Retention Strategies: Understanding behaviors can lead to enhanced retention strategies, driving sustained user engagement over time.
Lifecycle Stages and User Interactions
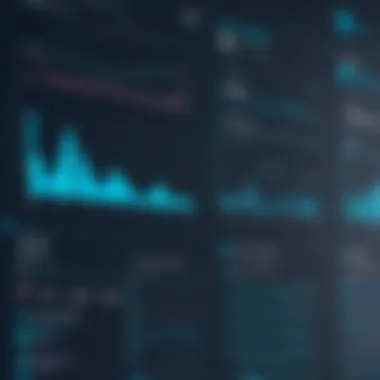
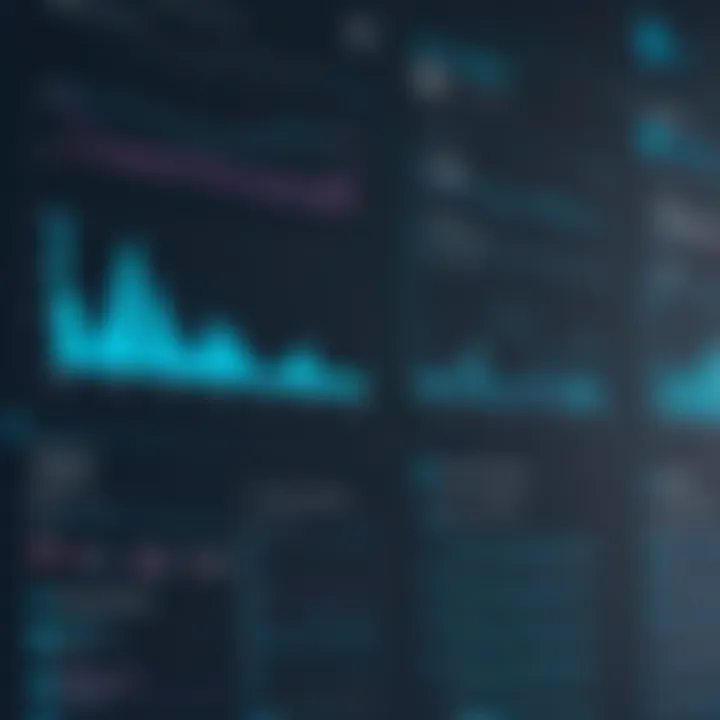
It’s essential to assess users based on their lifecycle stages. A new user has different needs and behaviors compared to a long-term customer. First impressions matter; therefore, how your software welcomes a newbie can dictate whether they stick around or bail out early. On another hand, loyal customers may require ongoing engagement strategies to maintain their interest.
- Important Aspects to Consider:
- Onboarding Processes: Cater onboarding experiences specifically for new users to get them acquainted effectively.
- Feedback Loops: Create opportunities for long-term users to provide input on features and updates, fostering a sense of ownership and loyalty.
- Usability Testing: Regularly examine how users at different stages are interacting with various features to ensure continual relevance.
"Understanding the nuances of user segmentation can greatly enhance how organizations approach their customers, focusing on needs and preferences rather than assumptions."
With a well-thought-out segmentation strategy in place, software professionals can work not just towards attracting users but also retaining them through a tailored and impactful experience.
Challenges in Behavioral Cohort Analysis
Understanding the challenges involved in behavioral cohort analysis is crucial for anyone looking to leverage user data effectively. As organizations increasingly turn towards data-driven strategies, the intricacies of this analysis become paramount. Behavioral cohort analysis can provide rich insights into user patterns, but several hurdles must be navigated for it to be truly effective. Key elements include the necessity to address data privacy, ensure data accuracy, and untangle the complexity of user behavior—all of which play a significant role in how findings can be useful and actionable.
Data Privacy Concerns
In today's digital landscape, data privacy is not just an afterthought; it's one of the pillars of user trust. When conducting behavioral cohort analysis, the handling of sensitive information can quickly become murky. Users are becoming increasingly aware of how their data is managed, and with regulations like GDPR gaining traction, companies have to tread carefully. Here are a few considerations:
- Consent: Users must give informed consent for their data to be used in cohort analysis. This necessitates clear communication about how their data will be employed.
- Anonymization: Anonymizing data helps mitigate risk, but it may also dilute the richness of insights. Striking a balance is essential.
- Data Breaches: Protecting against breaches isn't just about compliance; it's about preserving your organization's reputation. Any lapse could lead to loss of trust, which can ultimately cost businesses significantly.
"Trust is like a mirror; once it’s broken, it may be repaired, but it will never be the same."
Data Accuracy and Reliability
Without reliable data, any analysis falls flat on its face. The accuracy of data used in behavioral cohort analysis is paramount for sound outcomes. If the dataset is flawed, the insights gleaned may lead organizations down the rabbit hole of poor decision-making. Key points to keep in mind include:
- Source Validity: Ensure that the data comes from reputable sources. Poor quality data can skew results and render them useless.
- Data Cleaning: Regularly cleaning and updating datasets is crucial to maintain the reliability of your findings. Outdated or erroneous data can lead to misinterpretations.
- Metrics Definition: Clearly defining what metrics are being examined adds a layer of consistency. For instance, tracking user engagement could differ widely depending on whether you're measuring active sessions or account creations.
Complexity of User Behavior Patterns
User behavior is inherently complex, influenced by a myriad of factors ranging from social influences to individual preferences. This complexity can create a tangled web that poses significant challenges:
- Multi-faceted Influences: Users are not just one-dimensional; their behaviors are shaped by a series of interactions across various platforms. Understanding these can be like deciphering a puzzle where pieces are missing.
- Changing Trends: Trends can change overnight, especially in tech. A cohort analysis taken today might be less relevant in a month, necessitating ongoing adjustments.
- Interpreting Data: Raw data points can be misleading if not interpreted correctly. The challenge lies in distinguishing between correlation and causation; just because two variables seem linked doesn’t mean one causes the other.
In summary, behavioral cohort analysis carries its set of challenges that IT professionals, software developers, and businesses must grapple with. It's imperative to approach these challenges head-on while keeping the ultimate goal in mind: enhancing user experience and engagement through data-driven insights.
Best Practices for Implementing Behavioral Cohort Analysis
Integrating behavioral cohort analysis into a strategy can reap significant rewards for businesses wanting to understand their users better. It provides insights that help tailor products and marketing strategies, ultimately leading to heightened user satisfaction. To tap into the full potential of this approach, it's essential to follow certain best practices.
Establishing Clear Objectives
Before diving into any analytical undertaking, organizations ought to set clear objectives. Without knowing what you aim to achieve, it is easy to meander through data without any real direction. When forming objectives, consider what specific behaviors or trends you wish to analyze. Are you looking to increase user retention rates? Or perhaps your focus is on boosting engagement during a trial period? Whatever the case may be, outlining precise goals will streamline your efforts, enabling the selection of the right data to support these aims.
It's also critical to ensure that these objectives are measurable. Having quantifiable metrics allows one to assess the success of the implemented strategies. For example, if the objective is decreasing churn, tracking user activity and retention percentages is essential.
Using Correct Analytical Frameworks
Selecting the appropriate analytical frameworks plays a crucial role in effective cohort analysis. There are numerous tools available—ranging from spreadsheet applications to more sophisticated business intelligence software. The choice often hinges on the volume of data and the complexity of the analysis required.
Using frameworks that align well with your objectives can yield more accurate insights. For example, if your analysis focuses on time-series data, consider leveraging advanced modeling techniques like regression analysis or time-travel techniques to unveil underlying trends. On the other hand, if you’re interested in behavioral patterns, clustering techniques could help in categorizing users based on similar traits.
It's vital to remain adaptable in your choice of framework, as analytical needs might evolve with new data or changes in user behavior. Don't get stuck in a one-size-fits-all mentality; flexibility can often uncover insights you may have otherwise overlooked.
Continuous Monitoring and Adaptation
Engaging in behavioral cohort analysis isn’t a one-off task; it’s an ongoing exercise. Continuous monitoring of user behavior patterns allows for the identification of new trends and anomalies. By regularly analyzing data, organizations can stay ahead of shifts in user preferences or market conditions.
Moreover, having a flexible approach toward ongoing analysis can lead to better decision-making. When a new segment of users emerges, or an existing cohort behaves differently, organizations should be ready to adapt strategies accordingly. This adaptability fosters resilience and responsiveness, which are key traits of successful businesses today.
“In the world of analytics, stagnation is the enemy of progress. Continuous evaluation helps tackle new challenges as they arise.”
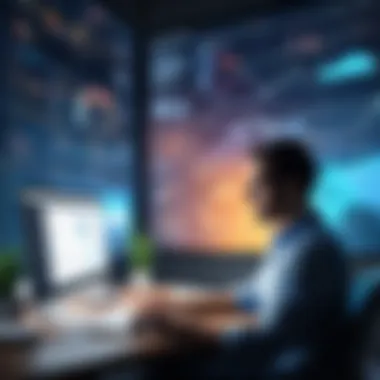
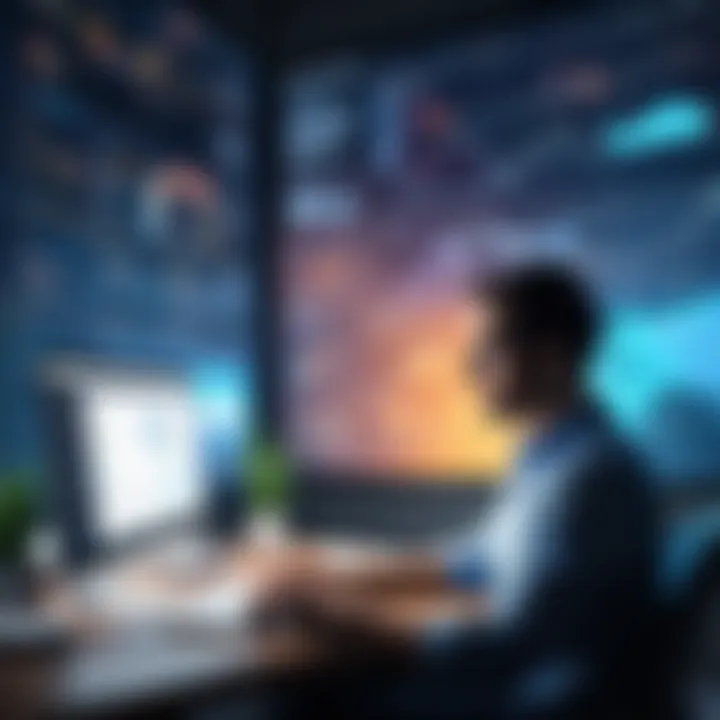
By implementing these best practices, organizations can position themselves for success in understanding user behavior more profoundly. Clear objectives, effective analytical methods, and ongoing monitoring not only ensure that insights are actionable but also make them relevant and timely in an ever-evolving landscape. With a solid foundation, businesses can draw on the fruits of behavioral cohort analysis to drive strategic decisions and enhance overall user satisfaction.
Real-World Case Studies
Exploring real-world case studies in behavioral cohort analysis provides valuable insights into how organizations apply this methodology effectively. These examples serve as beacons, illuminating paths with proven outcomes and strategies. When businesses examine specific instances of successful and unsuccessful cohort analysis implementations, it not only aids in understanding best practices but also highlights potential pitfalls that organizations should steer clear of.
Through real-world applications, various industries have embraced behavioral cohort analysis to enhance decision-making processes and refine user engagement strategies. Companies can see firsthand how cohort analysis informs product design, marketing tactics, and user retention methods, making these investigations crucial for those seeking a competitive edge in the market.
"Real-world case studies act as a mirror, reflecting both triumphs and challenges, teaching organizations the art of nuance in application."
Successful Applications of Cohort Analysis
Successful applications of cohort analysis paint a picture of how this methodology can transform business outcomes. For instance, a prominent travel booking site utilized cohort analysis to dissect user behavior pre- and post-booking. By segmenting users based on their booking timelines and engagement levels, the platform could tailor its marketing campaigns.
- Targeted Promotions: Groups characterized by high booking frequency received flash discounts, turning them into repeat customers. In contrast, users who abandoned bookings were engaged with tailored reminders and incentives tailored to entice them back.
- Enhanced User Interface: Insights gained from user cohorts allowed them to refine their website’s layout for a smoother booking process. They identified which features were often ignored and which were deemed essential. This refinement dramatically increased their conversion rates.
- Strategic Partnerships: Similarly, an e-commerce giant employed behavioral cohort analysis to refine its partnerships. By concentrating on users who frequented certain product categories, they could form alliances with related brands, creating bundle offers that drove higher sales.
These examples illustrate not just the feasibility but the necessity of coherent analysis within the realm of user behavior. Such approaches can lead companies to better maximize their marketing budgets while actually creating delightful user experiences.
Lessons Learned from Poor Implementations
Not every endeavor in behavioral cohort analysis leads to glowing success. Mishaps can offer significant lessons, throwing light on critical aspects that organizations must consider.
- Inadequate Data Quality:
In one case, a tech startup jumped straight into segmenting user data without establishing a clear data cleansing process. Their loss was glaring when they quickly learned that utilizing incomplete data would yield faulty insights, leading to misguided marketing strategies and wasted resources. - Ignoring User Feedback: Another crucial misstep occurred when a gaming company measured engagement solely through quantitative metrics, neglecting qualitative data. Feedback from users indicated that certain features were problematic, contrary to their data-driven assumptions. This oversight led the company to miss crucial improvements that could have retained users.
- Failure to Adapt: Moreover, sticking rigidly to a defined cohort without revisiting or adapting criteria is a common error. A retail business, for instance, failed to update its cohorts determined during holiday seasons, overlooking significant behavioral changes during other times of the year. The set-it-and-forget-it mentality hampered their ability to respond effectively to shifting user behaviors.
In wrapping up these lessons, it's evident that thorough preparation and continuous adaptation of strategies are vital. Organizations must cultivate a culture that values both data and user perspectives.
While successful applications showcase the positive impact of behavioral cohort analysis, poor experiences deliver a stark reminder of what not to do.
Future Trends in Behavioral Cohort Analysis
The field of behavioral cohort analysis is evolving rapidly, with emerging trends set to reshape how organizations approach and utilize user data. As technology continues to advance, the integration of more sophisticated tools becomes essential for businesses aiming to stay ahead in a competitive marketplace. By looking at future trends, we can uncover the significant benefits and considerations that will guide software evaluation and user engagement strategies.
Integration with Machine Learning
Machine learning is not just a buzzword; it's a game changer in the landscape of behavioral cohort analysis. As companies amass data, the need for more refined methodologies becomes clear. Machine learning algorithms can sift through vast amounts of user data much faster than traditional methods. This enhances the segmentation process, allowing analysts to identify cohorts based on nuanced behavioral patterns rather than broad, overlook generalizations.
For instance, businesses can employ clustering algorithms to differentiate between users who browse for specific interests but don't buy versus those who quickly make purchases. Using models like decision trees or neural networks, organizations gain insights into predictive behaviors that help concern themselves with potential drop-off points in the user journey.
Moreover, machine learning allows for continual learning and adaptation. Unlike fixed models, a well-implemented machine learning system can adjust predictions based on real-time engagement data. This not only improves accuracy but also builds a more dynamic understanding of user cohorts as their preferences shift over time. The predictive capabilities thus empower strategic decision-making and enhance user interactions with personalized experiences tailored to individual behaviors.
Impact of Emerging Technologies on Analysis
The swift pace of technological innovation brings numerous tools into the realm of behavioral cohort analysis. Technologies such as Artificial Intelligence (AI), the Internet of Things (IoT), and big data analytics produce extensive streams of data, leading to an abundance of information about user behavior. But more data doesn’t always equal better insights without the right analytical frameworks.
Key impacts include:
- Real-Time Data Processing: With systems that process data in real time, businesses can assess user behavior as it happens, allowing for timely interventions and personalized marketing efforts that resonate with users immediately.
- Cross-Platform Analytics: The convergence of various digital platforms means users interact with brands in multiple ways. Ensuring a cohesive understanding of these interactions helps in forming a holistic view of the user experience.
- Augmented Analytics: Automation tools now enable analysts to interpret data through visualization and natural language processing. This democratizes data insights, making it accessible for non-technical stakeholders to grasp user behavior trends effectively.
Emerging technologies are revolutionizing how we analyze user behavior. Incorporating these tools into behavioral cohort analysis not only improves precision but also elevates the entire experience for users and businesses alike.
"The integration of emerging technology in behavioral cohort analysis is not just about enhanced efficiency; it’s about creating deeper connections with users through informed understanding."
By committing to keeping pace with these trends, organizations can better position themselves to adapt and thrive in an evolving landscape, ensuring that they continue to meet the needs of their users while maximizing their operational potential.
Ending
Behavioral cohort analysis represents a pivotal approach in understanding user dynamics in a detailed way. It encapsulates the journey of users, breaking down their interactions into manageable segments that unveil underlying patterns. By navigating the intricacies of this analysis, organizations can not only tailor their offerings but also improve stakeholder relationships. The real strength lies in its ability to drive decision-making with informed insights, ultimately enhancing user satisfaction and retention.
Summary of Key Insights
In wrapping up the discussion on behavioral cohort analysis, a few key takeaways emerge:
- User Segmentation: The ability to dissect users into behavioral cohorts allows for targeted strategies that resonate with specific groups. This ensures that marketing and product development efforts have a higher success rate.
- Data-Driven Decisions: Using behavioral insights, companies can steer clear of guesswork. This analytical approach offers a robust foundation for strategic planning, minimizing risks associated with misaligned products or services.
- Enhanced User Experience: By understanding user journeys and preferences, organizations can refine their platforms, leading to smoother interactions. This is not just about making things prettier; it’s about making things more functional and accessible.
- Adaptability: The continuous nature of behavioral cohort analysis promotes a cycle of adaptation. As user behaviors evolve, the insights derived from ongoing analysis equip businesses to pivot when necessary, ensuring they remain relevant and competitive.
The Road Ahead for Software Evaluation
Looking ahead, the landscape of software evaluation is bound to get more sophisticated. With advancements in technology, particularly in areas like artificial intelligence and machine learning, the integration of these tools will redefine how behavioral cohort analysis is executed.
- Predictive Analytics: Future methodologies will likely lean on predictive analytics. Harnessing data from behavioral patterns not only assists companies in understanding past interactions but also equips them to forecast future user behaviors.
- Augmentation through AI: Automated tools will emerge that can handle vast amounts of data with precision, potentially allowing businesses to focus on broader strategy matters instead of getting bogged down in minutiae. Tools that can learn from user data patterns will become invaluable assets.
- Holistic Insights Across Platforms: As businesses become more digital, gathering insights across various platforms will be critical. Behavioral cohort analysis can expand beyond standalone software products, factoring in interactions across social media, mobile applications, and web platforms to get a more rounded picture.
- Emphasis on Ethical Data Use: With a rising awareness of data privacy, the methods employed in behavioral cohort analysis must align with ethical standards. Organizations need to ensure user trust while still gaining valuable insights, a balancing act that will define future practices.
The journey of behavioral cohort analysis continues to evolve. Organizations that grasp its importance and strategically implement its practices stand to gain a significant edge in understanding and serving their users.