Exploring Data Analytics and Reporting Tools
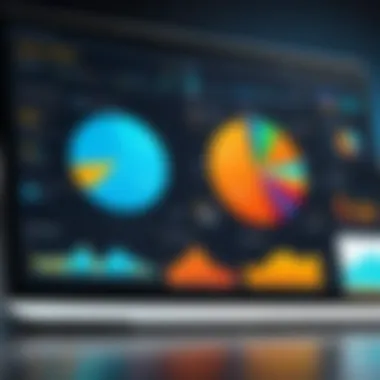
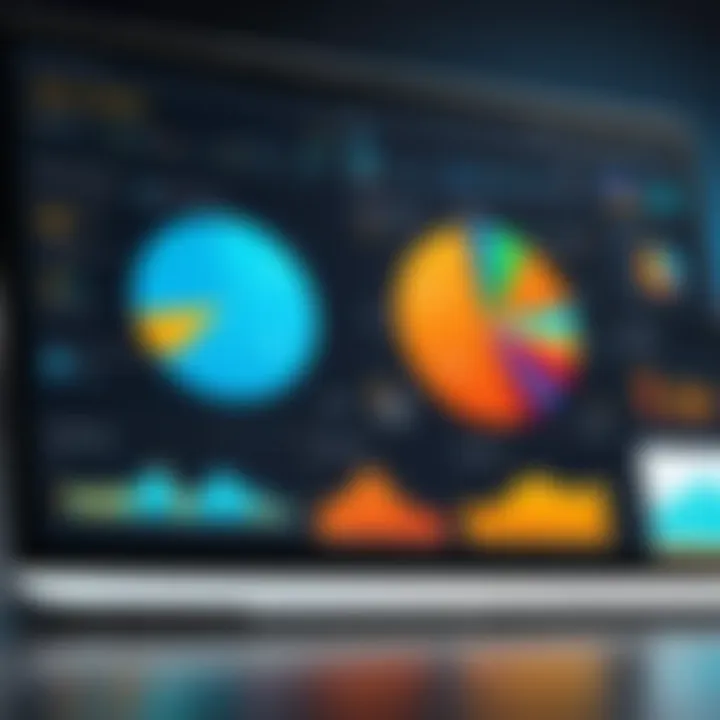
Intro
In today's data-driven world, the ability to analyze and report on vast amounts of information is pivotal. Organizations of all sizes benefit from insights gained through effective data analytics and reporting tools. These tools are no longer just optional; they are essential for informed decision-making. With the rise of complex data environments, understanding the functionalities and applications of these tools can significantly enhance strategic initiatives.
Data analytics encompasses a wide range of techniques that can transform raw data into meaningful insights. Reporting tools complement this process by presenting these insights in a clear and accessible manner. Professionals need to navigate the multitude of available tools, focusing on those that align with their specific requirements. This article will offer an in-depth look at the key aspects of data analytics and reporting tools, paving the way for more effective data-driven decisions across various sectors.
Software Overview
Software Description
Data analytics and reporting software are designed to facilitate the collection, processing, and visualization of data. These tools cater to varying levels of analytical sophistication, from basic descriptive analytics to more advanced predictive modeling and machine learning capabilities.
Among the popular tools in this category are Tableau, Power BI, and Google Data Studio. Each of these offers unique features and strengths, addressing different needs in the market. For example, Tableau excels in data visualization, while Power BI provides seamless integration with Microsoft products, and Google Data Studio shines in accessibility and collaboration capabilities.
Key Features
Several key features define effective data analytics and reporting tools:
- Data Connectivity: Ability to connect to diverse data sources, such as databases, cloud services, and spreadsheets.
- Visualization Capabilities: Robust options for creating various types of graphs, charts, and dashboards.
- Collaboration Tools: Features that promote sharing insights among team members and stakeholders.
- Real-time Analytics: Support for live data connections, enabling immediate insights.
- User Customization: Options for users to tailor reporting formats and analytics to specific needs.
These features collectively enhance the usability and effectiveness of these tools, allowing professionals to derive meaningful insights with ease.
User Experience
User Interface and Design
A user-friendly interface is crucial in data analytics and reporting tools. It impacts how efficiently professionals can navigate the software and derive insights. Tools like Power BI are known for their intuitive design, which helps users with varying technical skills get started quickly.
The layout typically includes drag-and-drop functionality, enabling users to create reports dynamically without extensive training. Good design is not merely aesthetic; it plays a fundamental role in user engagement and productivity.
Performance and Reliability
Performance is another critical consideration. High-performance analytics tools can handle large volumes of data without lagging, ensuring timely analyses. Reliability refers to the software's consistency in delivering accurate results and maintaining uptime. Organizations often favor tools with a strong track record in both performance and reliability, as these factors are essential for maintaining trust in the insights they produce.
"The right data analytics solutions can empower organizations to make informed decisions, driving growth and efficiency across all sectors."
Understanding Data Analytics
Understanding data analytics is pivotal for organizations striving to harness data's potential. In today's data-driven landscape, the ability to analyze and interpret data informs decision-making processes across all levels of business. Effective data analytics leads to actionable insights that can enhance performance, improve customer satisfaction, and identify new opportunities.
Data analytics involves collecting, processing, and analyzing data to extract meaningful patterns and insights. Leaders in various sectors apply these insights to increase efficiency, mitigate risks, and drive growth. It is no longer merely a technical function; it plays a critical role in shaping strategic direction.
Definition and Importance
Data analytics refers to the systematic analysis of datasets to uncover trends, correlations, or insights. It encompasses various tools and methods that help organizations understand their data.
The importance lies in its ability to transform raw data into actionable insights. With effective data analytics, organizations can anticipate customer needs, streamline operations, and make informed strategic decisions.
In essence, data analytics enables businesses to remain competitive and adapt to changing market conditions.
Types of Data Analytics
Understanding the various types of data analytics is crucial for selecting the appropriate approach for decision-making. The three main types are:
Descriptive Analytics
Descriptive analytics focuses on summarizing historical data to reveal trends and patterns. It answers the question, "What happened?" This type utilizes various statistical measures, such as mean, median, and mode, to present a clear picture of past events.
A key characteristic of descriptive analytics is its use of data visualization tools, such as charts and graphs, to present data in an understandable format. This makes it a popular choice for businesses seeking to gain insights into their past performance and identify areas for improvement.
One unique feature of this type is its ability to provide a retrospective view, which aids organizations in formulation of evidence-based strategies. However, it does not predict future outcomes, which might be seen as a limitation.
Predictive Analytics
Predictive analytics, in contrast, attempts to forecast future events based on historical data set. It uses statistical algorithms and machine learning techniques to identify potential trends.
The key characteristic of predictive analytics is its focus on probabilities. By examining historical data, it can predict future behavior and events, which is invaluable in areas such as marketing and risk management.
A unique feature of predictive analytics is its capability to aid decision-makers in resource allocation. This can enhance efficiency and effectiveness in operations. However, the quality of its predictions largely depends on the quality of the input data and the chosen predictive model.
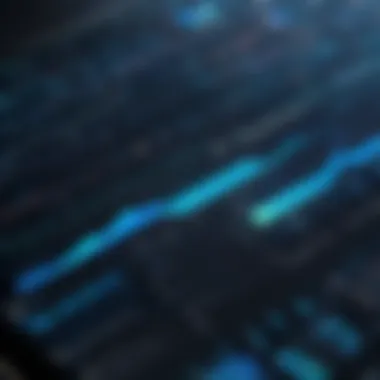
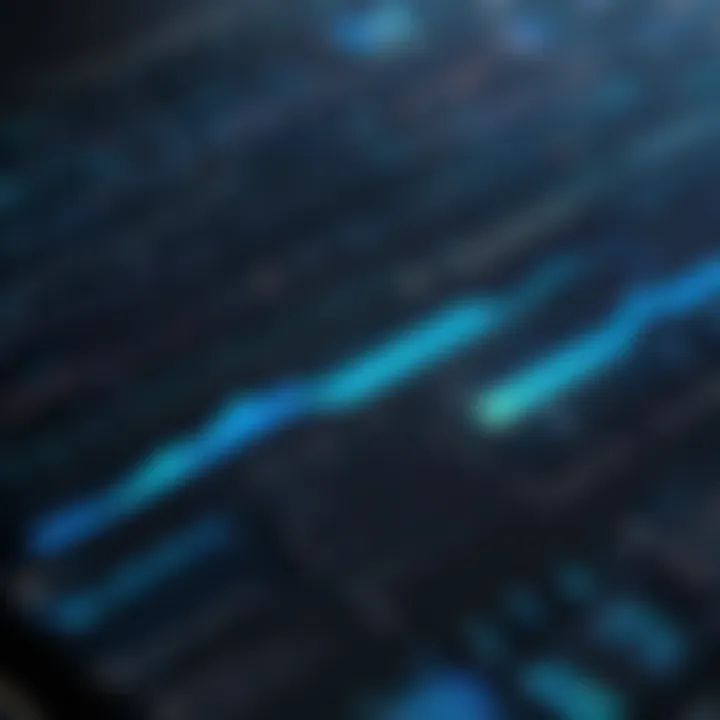
Prescriptive Analytics
Prescriptive analytics takes the process a step further by recommending actions based on the analysis of data. It answers the question, "What should we do?" This involves not only predicting outcomes but also suggesting the most effective strategies to achieve desired results.
Its key characteristic is its use of algorithms to evaluate various options and their potential outcomes. This makes it a powerful tool for organizations looking to optimize processes and decisions.
One notable feature of prescriptive analytics is its ability to offer real-time suggestions based on current data. This can lead to quick, informed decision-making. On the downside, the complexity of implementing prescriptive analytics can be a barrier for some organizations.
The Role of Reporting Tools in Data Visualization
Reporting tools play a crucial role in data visualization by transforming raw data into comprehensible formats. These tools facilitate the analysis and interpretation of data, making complex insights accessible to various stakeholders. The significance of reporting tools lies not only in their ability to present data visually but also in their capacity to enhance decision-making processes within organizations.
Data visualization is inherently about revealing patterns, trends, and insights from data. Reporting tools, therefore, act as essential conduits through which this data becomes understandable. A well-designed reporting tool allows users to navigate through vast datasets, enabling them to focus on key metrics and draw meaningful conclusions.
Key Functions of Reporting Tools
Reporting tools offer several critical functions that contribute to effective data visualization:
- Data Consolidation: They aggregate information from multiple sources and present it in a unified view. This consolidation helps eliminate silos and promotes a holistic understanding of the organization's data landscape.
- Visualization Options: These tools provide various visualization formats, such as charts, graphs, and dashboards, allowing users to choose the most effective method to represent data for their needs. The choice of visualization can greatly impact how insights are understood.
- Interactivity: Many reporting tools offer interactive features, enabling users to explore data dynamically. This interactivity fosters a deeper engagement with the data and enhances the overall analytical experience.
- Customization: Most reporting tools allow users to customize dashboards and reports. Customization ensures that reports align with specific business needs and address particular analytical requirements.
Moreover, reporting tools are not just designed for data analysts. They cater to a wide audience, including IT professionals and business executives. This versatility enhances their importance in todayโs data-driven environment.
Integration with Data Sources
Integration is one of the most critical aspects of effective reporting tools. These tools are designed to connect with various data sources seamlessly. This integration can include databases like MySQL, cloud sources such as Google Drive, or even APIs from other software applications.
- Real-Time Data Access: Integration enables real-time data retrieval, which is vital for businesses that rely on up-to-the-minute information. It allows for timely reporting and responsive decision-making.
- Flexibility: Reporting tools should support numerous data formats and types. This flexibility ensures that users can work with their preferred datasets, regardless of how they are stored.
- Data Transformation: Before visualization, data often requires transformation. Sophisticated reporting tools provide capabilities to clean, shape, and normalize data during the integration process. This functionality ensures the accuracy and relevance of the data presented.
Features of Effective Data Analytics Tools
In today's data-driven world, selecting the right data analytics tools is crucial for maximizing insights and informed decision-making. Different industries rely on specific features in these tools to meet their unique needs. Understanding these features helps organizations evaluate potential solutions that can lead to improved business outcomes.
User Interface Considerations
A well-designed user interface (UI) is essential for effective data analytics tools. The UI should be intuitive, enabling users to navigate quickly and easily. A clean layout reduces the learning curve for new users, making it easier for teams to adopt the tool. Features such as drag-and-drop functionalities, customizable dashboards, and clear visualizations enhance usability. Users should be able to find the information they need without confusion, leading to more efficient analysis and reporting. Furthermore, the tool should support multiple devices, ensuring access for users in various environments.
Data Integration Capabilities
Effective data analytics tools must seamlessly integrate with diverse data sources. Organizations often use multiple systems to collect and store data. Therefore, the ability to connect with databases like Microsoft SQL Server, NoSQL solutions such as MongoDB, or cloud services like Google Cloud Platform is vital. The integration capabilities should allow users to pull data from different platforms without requiring extensive technical knowledge. Users benefit from access to comprehensive datasets, enabling better analysis. Ensuring data quality throughout this integration process is also crucial. Users should verify that the data is accurate, consistent, and timely for informed decision-making.
Scalability and Flexibility
The landscape of data analytics is constantly changing. Thus, tools must be scalable and flexible to adapt to a company's growth. As businesses expand, they accumulate more data. Effective tools should handle this increasing data volume without performance issues. It's important that organizations choose tools that can scale, whether through cloud infrastructure or on-premises solutions. Additionally, flexibility is key in accommodating different data types and analysis methods. A tool that allows customization of functionalities will better serve a variable business landscape.
"To thrive in a competitive environment, organizations must leverage the right analytics tools that fit their specific needs and enhance their decision-making capabilities."
In summary, focusing on user interface design, data integration capabilities, and scalability ensures organizations can effectively utilize their data analytics tools. This ultimately supports better decision-making and improved outcomes in various sectors.
Evaluating Popular Data Analytics Tools
Evaluating popular data analytics tools is a crucial step for organizations seeking to leverage data for informed decision-making. These tools play an essential role in converting raw data into meaningful insights. With the vast array of options available, the evaluation process must focus on several key factors to ensure the selected tool aligns with specific business needs.
Choosing the right analytics tool can offer several benefits. It can streamline operations, enhance data visualization, and improve overall business intelligence efforts. A well-chosen tool can also lead to significant cost savings and improved productivity. Thus, to navigate through the multitude of options, it is important to assess these tools systematically.
Feature Comparison
When comparing features of data analytics tools, several criteria should be taken into account. Essential features include data visualization options, integration capabilities, and analytical functions. The user interface must also be considered, as a clean and intuitive design often leads to improved user experience.
Notably, it is useful to distinguish between different types of analytics functions offered. For example:
- Dashboards and Reports: Ensure that the tool allows easy creation and sharing of customizable dashboards and automated reports.
- Machine Learning: Some tools provide advanced functionalities such as predictive analytics, which can enhance forecasting abilities.
- Collaboration Features: Tools that allow team collaboration can help streamline processes and encourage collective analytical efforts.
Reviewing these features thoroughly aids organizations in selecting a tool that not only meets their immediate analytical requirements but also accommodates future growth.
Pricing Models
Pricing is a significant factor in tool evaluation. Different pricing models can impact the overall budget and resource allocation. Understanding the cost structure for each tool is vital.
Common pricing models include:
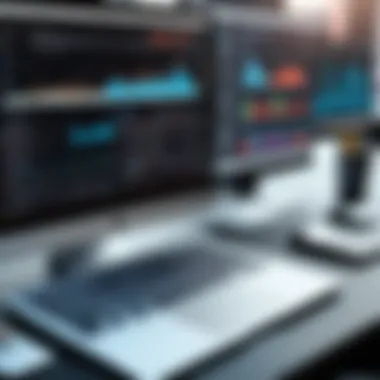
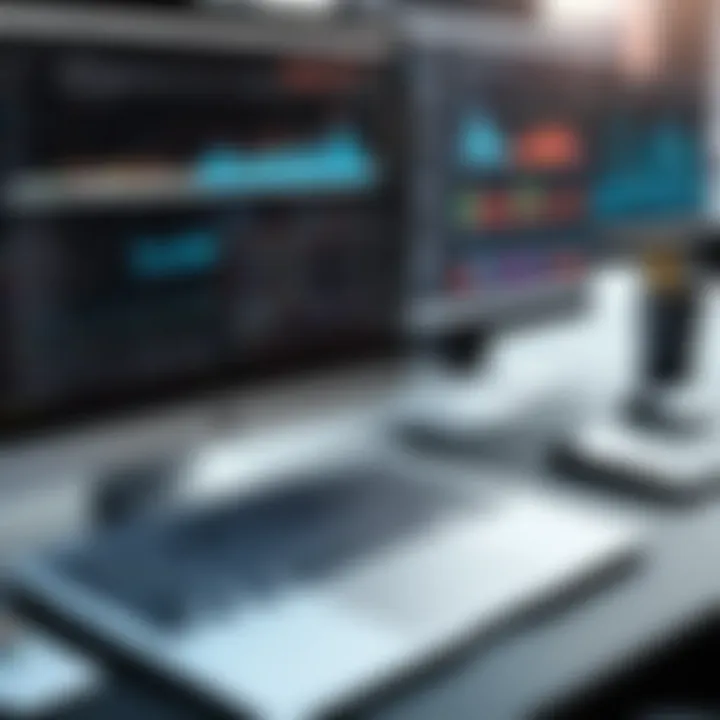
- Subscription-Based: Tools like Tableau and Microsoft Power BI often operate on a monthly subscription basis, allowing flexible access to services.
- One-Time License Fee: Some applications require a one-time purchase. This may be more cost-effective in the long run, particularly for larger organizations who can predict usage needs.
- Pay-as-You-Go: This model charges based on the volume of data processed or the number of users, which can be beneficial for companies with fluctuating analytics demands.
Carefully assessing how pricing aligns with features and user needs is key to ensuring the chosen tool represents good value.
User Feedback
User feedback is a valuable component in evaluating data analytics tools. Reviews from actual users can provide insights not captured during feature assessments. Feedback can highlight both strengths and weaknesses of a tool, informing potential buyers about real-world performance.
To gather user feedback effectively, consider checking:
- Online Reviews: Sites like Reddit or Trustpilot often have user-generated feedback that can be instrumental.
- Community Forums: Engaging with user communities can bring forth discussions about troubleshooting, updates, and overall satisfaction.
- Case Studies: Companies often publish case studies detailing their experience with a particular tool, demonstrating outcomes achieved through analytics use.
In summary, evaluating data analytics tools encompasses feature comparison, understanding pricing models, and reviewing user feedback. This thorough approach enables organizations to select tools that not only meet their analytical needs but also align with their strategic objectives. By focusing on these considerations, companies can make informed choices that enhance their decision-making processes.
Popular Data Analytics Tools Reviewed
The landscape of data analytics is rich and diverse, and choosing the right tools can significantly influence the quality of insights derived from data. Those tools are not just software; they represent essential aids for professionals aiming to make informed decisions. In this section, we will examine three prominent data analytics tools, analyzing their key features, pricing structures, and ideal use cases. Understanding these aspects can help businesses align their needs with the right analytic solutions.
Tool A Overview
Key Features
Tool A stands out primarily for its intuitive user interface and robust data processing capabilities. It simplifies complex datasets, enabling users to visualize information without needing extensive training. This feature is pivotal for teams that may not have specialized data skills. One distinct characteristic of Tool A is its algorithmic capabilities that enhance data forecasting accuracy. However, while these features render Tool A a popular option, its heavy reliance on the cloud may raise concerns about data security for privacy-sensitive organizations.
Pricing
The pricing model for Tool A is subscription-based, which makes it accessible for small to mid-sized enterprises. The tiered pricing allows users to scale their investment according to their specific needs. However, it's vital to note that frequent updates lead to price adjustments, which may surprise longtime users. This aspect can be beneficial for new users who are looking for versatility, though existing clients may find it challenging to manage budgets over time.
Best Use Cases
Tool A is particularly effective in the domains of retail analytics and customer behavior analysis. Its real-time processing capabilities make it suitable for businesses needing immediate insights into sales trends. On the downside, some users have reported limitations when applying Tool A for extensive historical data analysis, which could disadvantage industries that rely heavily on past performance metrics.
Tool B Overview
Key Features
Tool B is known for its strong integration with various data sources and tools. This feature allows seamless connectivity with popular databases and CRMs, enhancing the user experience. The versatility of Tool B, particularly in custom reporting options, is another strong point that positions it favorably for teams requiring tailored analysis. Nevertheless, integrating new data sources can sometimes be time-consuming, particularly for non-technical users.
Pricing
Tool B employs a pay-per-user pricing model, which could be advantageous for organizations that want to manage costs closely. Teams only pay for the users actively engaged with the software. However, this model can become costly if the user base expands significantly. The cost must be weighed against the flexibility offered, as it allows organizations to scale up or down based on project needs.
Best Use Cases
Tool B excels in sectors like financial services and healthcare, where compliance and precise data reporting are crucial. It is effective for generating detailed regulatory reports, which is invaluable for these industries. A challenge arises when organizations attempt to adapt Tool B for more creative functions, such as marketing analytics, where its traditional reporting capabilities may not satisfy expectations.
Tool Overview
Key Features
Tool C is characterized by advanced machine learning tools that provide predictive insights. It leverages modern algorithms to analyze trends and user behaviors. This feature is especially beneficial for organizations aiming to anticipate market shifts before they occur. However, the complexity associated with machine learning can intimidate some users unfamiliar with data science principles.
Pricing
The pricing structure for Tool C operates on a freemium model, permitting users to access fundamental features without cost. This model can attract organizations willing to explore before committing. However, unlocking advanced features requires an investment, which could deter companies with limited budgets in the initial phases of adoption.
Best Use Cases
Tool C is well-suited for businesses in fast-paced industries such as tech startups and online retail. Its predictive analysis capabilities allow these firms to adjust strategies proactively. However, its reliance on significant data inputs can pose challenges for smaller businesses lacking extensive datasets.
Case Studies Demonstrating Effective Use of Analytics
Case studies serve as powerful narratives that illustrate the practical applications of data analytics across various industries. They showcase real-world scenarios where organizations have successfully leveraged data insights to drive decision-making and operational improvements. By examining these case studies, IT professionals and business leaders can glean valuable lessons about best practices, potential pitfalls, and the transformative power of analytics. Additionally, these studies can highlight the specific needs of businesses and how tailored analytics solutions can address them.
Industry A Case Study
In the healthcare sector, the use of analytics has become increasingly vital for improving patient outcomes and optimizing operational efficiency. A prominent example is the case of Mount Sinai Health System in New York City. This institution implemented a predictive analytics framework to identify patients at risk of readmission after discharge. By analyzing previous hospitalization data, patient demographics, and treatment histories, the system developed predictive models that alerted healthcare providers to at-risk patients.
As a result, Mount Sinai was able to implement targeted intervention programs, such as tailored follow-up appointments and personalized care plans. The outcomes were promising, showing a significant reduction in readmission rates, which also contributed to cost savings for both the health system and patients. This case demonstrates how data analytics can lead to better patient care while simultaneously improving health system efficiency.
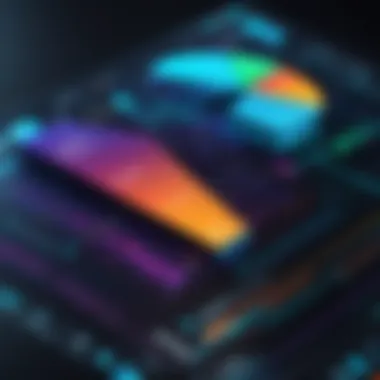
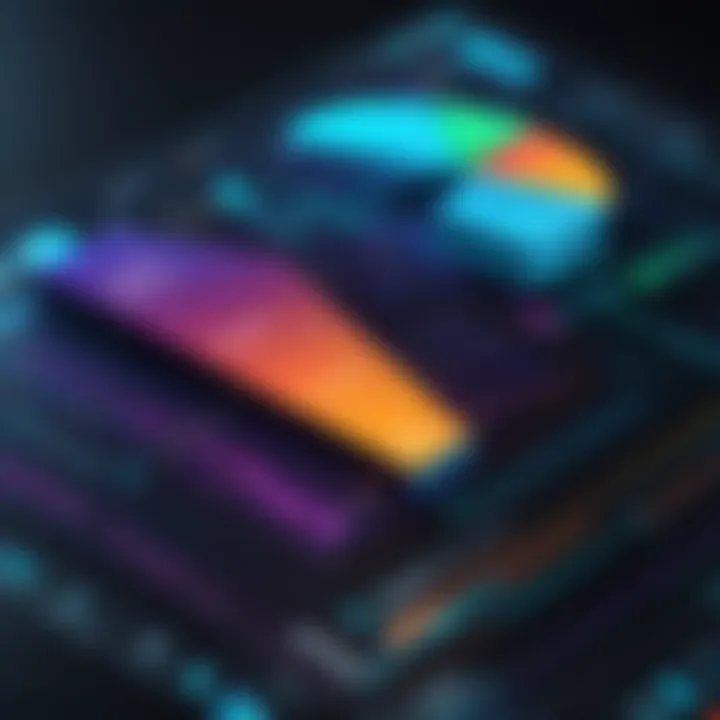
Industry B Case Study
In the retail sector, data analytics plays a critical role in enhancing customer experiences and optimizing inventory management. Target Corporation provides an illustrative case in this domain. The retail giant utilizes a robust analytics framework to understand customer purchasing behaviors and trends. By segmenting customer data, including buying patterns and preferences, Target can personalize marketing efforts and tailor promotions based on individual interests.
One noteworthy instance involved Target's ability to predict customer maternity needs based on changes in shopping habits. By analyzing the purchasing behavior of first-time mothers, Target successfully developed targeted marketing campaigns, offering promotions on baby-related products. The result was a measurable increase in sales from this demographic, ultimately reinforcing customer loyalty. This case underscores how analytics can drive better marketing strategies and enhance customer engagement in the retail environment.
Challenges in Data Analytics and Reporting
In today's data-driven world, challenges in data analytics and reporting can have significant implications for organizations. Understanding these challenges is crucial for IT and software professionals, as it impacts decision-making, strategy formulation, and ultimately, business success. By addressing these issues, companies can enhance their data analytics capabilities and ensure effective reporting outcomes.
Data Quality Issues
Data quality issues remain a persistent challenge in analytics. Poor quality data can lead to erroneous conclusions and misguided business strategies. Factors contributing to these issues often include:
- Incomplete Data: Missing data points can distort overall analysis.
- Inaccurate Data: Errors during data entry or processing lead to misinformation.
- Data Duplication: Redundant data can skew results and analysis.
- Outdated Information: Using old datasets can hinder the relevancy of insights.
To mitigate these data quality issues, organizations need to implement strong data governance practices. Regular audits, validation, and cleansing processes help maintain data integrity. Additionally, utilizing tools that automatically flag inconsistencies can significantly reduce the risk of utilizing faulty data in analysis and reporting.
"The quality of your data directly influences the quality of your insights."
Understanding User Needs
Another challenge is understanding user needs accurately. Data analytics tools will only be effective if they align with the specific requirements of various stakeholders. Misalignment can lead to wasted resources and unmet objectives. Key considerations in this area include:
- Identifying Stakeholders: Recognizing who will use the analytics is essential. Different roles may require distinct data presentations or metrics.
- Defining Objectives: Setting clear goals helps to tailor analytics processes to meet specific outcomes.
- User Training: Providing adequate training ensures that users understand how to extract insights effectively from analytics tools.
Gathering feedback from users during tool implementation can greatly assist organizations. Engaging users throughout the process allows for better understanding of their needs and preferences, ensuring that the selected tools deliver actionable insights.
Overall, recognizing these challenges provides an opportunity for organizations to strengthen their approach to data analytics and reporting, thus enhancing the overall effectiveness of their data-driven strategies.
Future Trends in Data Analytics
Understanding future trends in data analytics is key for any organization looking to stay competitive. The rapid pace of technological advancement continuously reshapes the landscape of data utilization. As decision-making increasingly leans on data insights, comprehending these trends is not just important but necessary for success. Organizations that can adeptly navigate this evolving terrain stand to gain significant advantages over their peers. The exploration of artificial intelligence, real-time analytics, and data democratization reveals how these components can dramatically transform data strategies.
Artificial Intelligence in Analytics
Artificial Intelligence (AI) is becoming a cornerstone in data analytics. Its application enhances pattern recognition, forecasts, and decision-making processes. AI systems can analyze vast amounts of data more quickly than humans, uncovering insights that might otherwise be missed. Machine learning models enable predictive analytics that can forecast trends based on historical data and real-time inputs. This ability not only boosts precision but also accelerates response times to market changes.
Incorporating AI into data analytics can lead to substantial cost savings and efficiency improvements. Companies can automate routine analyses, freeing up human resources for more strategic functions. As AI continues to evolve, we can expect more sophisticated algorithms and tools designed specifically for varied industrial needs.
Real-Time Analytics
The advent of real-time analytics is reshaping how organizations respond to data. Unlike traditional analytics that often operate on historical data, real-time analytics processes data as it is created. This capability offers companies immediate insights, allowing for timely decision-making. In sectors like e-commerce, finance, and healthcare, the significance of real-time data cannot be understated.
With real-time analytics, businesses can monitor and react to customer behavior instantaneously. This ability fosters improved service and enhances customer experience. Moreover, it enables organizations to promptly identify and mitigate risks, thereby improving operational resilience and agility. As technology advances, the demand for real-time analytics will likely grow, pushing companies to adapt or risk obsolescence.
Emergence of Data Democratization
Data democratization is a compelling trend, aimed at making data accessible to non-technical stakeholders. Instead of a select few having exclusive access to data, organizations are focusing on empowering all employees with data insights. This shift enhances collaboration, promotes data-driven decision-making, and fosters a culture of innovation.
Practically, data democratization can involve deploying user-friendly tools that allow employees to gather insights without needing deep analytical skills. Tools like Tableau and Microsoft Power BI enable this accessibility. As a result, organizations may realize improved employee engagement and enhanced decision quality. However, implementing data democratization also requires a cultural shift and a commitment to data governance. This ensures that all employees can access data responsibly and ethically.
"Data democratization not only transforms how decisions are made but also nurtures a more informed workforce, leading to overall organizational growth."
In summary, understanding and adapting to these future trends is essential. Organizations aiming to thrive in a data-driven world need to embrace AI, leverage real-time analytics, and foster a culture of data democratization. These elements are not only beneficial but also critical as data becomes an even more integral part of strategic planning and execution.
Best Practices for Implementing Analytics Tools
Implementing analytics tools effectively is crucial for maximizing the value of data within an organization. Best practices ensure that these tools align with organizational objectives and deliver actionable insights. Organizations can avoid common pitfalls like poor adoption rates or misaligned goals by following specific strategies. This section outlines the importance of setting clear objectives and facilitating user training, as they are critical for successful implementation.
Setting Clear Objectives
Establishing clear objectives is the starting point of any analytics tool implementation. It is essential to determine what the organization hopes to achieve through data analytics. This clarity in purpose guides all subsequent actions, from tool selection to data analysis strategies. Tight alignment with business strategies helps in identifying necessary data sources and determining the metrics that matter most to stakeholders.
Without defined goals, the implementation may lead to inefficiencies, wasted resources, and confusion. Here are some key points to consider when setting objectives:
- Specificity: Objectives should be precise. For example, rather than aiming for "better customer insights," a more specific goal could be "increase customer retention by 15% over the next quarter."
- Measurability: Establish measurable criteria to assess the effectiveness of analytics efforts. This aspect helps in evaluating progress and making necessary adjustments.
- Relevance: Ensure that the defined objectives are relevant to the broader organizational strategy. This alignment secures buy-in from leadership and stakeholders.
- Timeliness: Set a timeline that outlines when objectives should be met. This structure promotes accountability among team members.
"Clear, actionable objectives are the backbone of successful data analytics implementations. Without them, all technical efforts may lead to ambiguity and disillusionment."
Training and User Acceptance
Training is another critical component of successfully implementing analytics tools. Even the most sophisticated tool is useless if end-users do not understand how to utilize it effectively. Fostering user acceptance through tailored training programs is essential for ensuring that team members can leverage analytics to inform their decisions. Here are some strategies to promote training and acceptance:
- User-Centric Design: Tools should be intuitive. Designers and IT teams should consider user feedback to improve functionality and usability.
- Comprehensive Training Programs: Offering a range of training sessionsโsuch as workshops, online courses, and one-on-one coachingโcan accommodate varying levels of expertise among users.
- Involvement from the Start: Engage users early in the process. Understanding their needs can guide not only the choice of tools but also the training approach.
- Support Channels: Create support resources like help desks or forums. Users should have places to seek help, share experiences, and discuss challenges they face.
Overall, the success of analytics tools in driving impactful insights relies not just on the technology but also on how well the users adapt. Bringing clarity to goals combined with robust user training creates an environment ripe for informed decision-making.