Mastering the Steps of Master Data Management
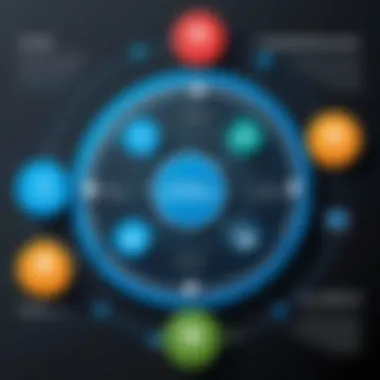
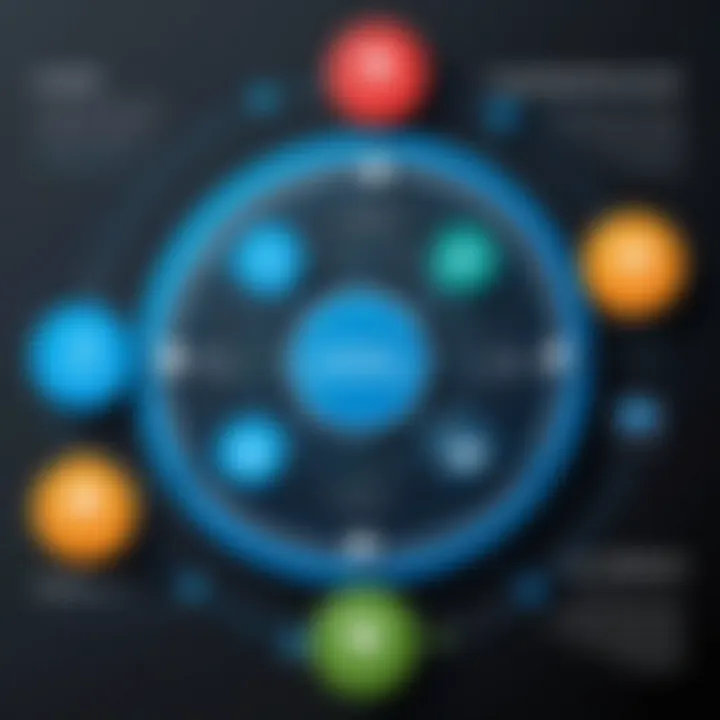
Intro
Master Data Management (MDM) represents a pivotal function in the landscape of modern business operations. In today's world, organizations strive for data integrity, accuracy and the seamless flow of information. This is where MDM steps into the limelight, acting as the backbone of effective decision-making processes. Moreover, with the vast amounts of data generated daily, it becomes paramount for companiesâboth big and smallâto ge their arms around what master data is and how to handle it efficiently.
Understanding MDM can be compared to piecing together a jigsaw puzzle. Each piece of data must fit perfectly within the larger framework, ensuring that decision-makers have a clear and comprehensive view of the information at hand. In this guide, we delve not only into the theoretical underpinnings of MDM but also unpack its critical stages and practical applications, ultimately arming software professionals and key decision-makers with actionable insights for successful implementation.
As we move through the subsequent sections, readers can anticipate an exploration into various methodologies, benefits, challenges, and the tools that facilitate effective MDM. From the inception of data governance policies to the ongoing processes of data consolidation, this comprehensive narrative seeks to illuminate the intricate steps and best practices needed to maintain an authoritative data repository.
Understanding Master Data Management
Master Data Management (MDM) is pivotal in todayâs data-driven world. With organizations inundated with a vast array of data, MDM serves as a critical strategy for businesses looking to maintain data integrity and streamline operations. Essentially, MDM is about ensuring that all data across an organization is accurate, consistent, and accessible. But it isnât just a tech issue; itâs deeply woven into the fabric of organizational success.
By effectively implementing MDM, businesses can expect to see a range of benefits. Not only does it help in mitigating risks associated with poor data governance, but it also lays the groundwork for bolstering customer relationships through reliable data. When leaders understand the importance of clean, coherent master data, they create a solid base for making strategic decisions.
However, understanding MDM requires a consideration of different dimensions, which becomes apparent when we break it down into specific areas. Letâs dig deeper.
Definition of Master Data Management
Master Data Management refers to the processes, governance, and tools that organizations use to create and maintain a single, accurate view of their critical business data. Think of it as a central hub where all essential data elementsâsuch as customer information, product details, and vendor recordsâare consolidated and consistently curated.
Itâs not merely about data storage; itâs about data stewardship. Without an effective MDM strategy, organizations face chaos and duplication of data, which can lead to errors in operations and poor decision making.
Significance of in Modern Organizations
MDM is indisputably significant in modern environments where data acts as a cornerstone of operational efficacy and strategic advantage. It transforms raw data into reliable insights. For instance, consider a retail giant: with MDM, it can integrate its customer data from in-store purchases with online shopping habits. By doing this, it understands customer behaviors more thoroughly and can tailor marketing strategies accordingly.
Moreover, organizations that prioritize MDM enhance compliance with regulations like GDPR or CCPA, steering clear of potential legal issues associated with data mishandling.
"In an age where data is often referred to as the new oil, MDM helps refine that oil into valuable insights for better decision-making."
Components of
At its core, MDM encompasses several crucial components that ensure effective data management. These include:
- Data Governance: Establishing rules, processes, and standards for managing master data is essential. Without it, you risk allowing data inconsistencies to creep in.
- Data Quality: Ensuring that data is not just accurate but also relevant and timely is paramount. Clean data breeds confidence in decision-making.
- Data Integration: Aligning disparate data sources through integration enables a holistic view of information across systems.
- Data Models: Creating clear data models that define how data elements relate can simplify management processes.
Each of these components plays a vital role in ensuring that MDM is not just a statement of intent, but an actionable framework.
Common Challenges in Implementation
Implementing MDM is not without its hurdles; understanding these common challenges can sometimes be half the battle. Organizations grapple with various issues, such as:
- Cultural Resistance: Data governance may be met with skepticism among employees who fear that new protocols could bind them to practices they find cumbersome.
- Complexity of Data Sources: A business might operate with multiple legacy systems that hold different versions of the same data, leading to confusion and inconsistency.
- Resource Constraints: Often, MDM projects battle for attention and resources against other pressing operational needs.
- Defining Ownership: Determining who owns which data element can become a thorny issue, with stakeholders often at odds.
Navigating these challenges requires careful planning and communication, ensuring that all involved understand the purpose and benefits of MDM.
In summary, understanding Master Data Management is foundational for any organization aiming for operational excellence. The merits of maintaining clean, integrated master data echo throughout all facets of business decision-making, ultimately steering organizations toward success.
Key Steps in
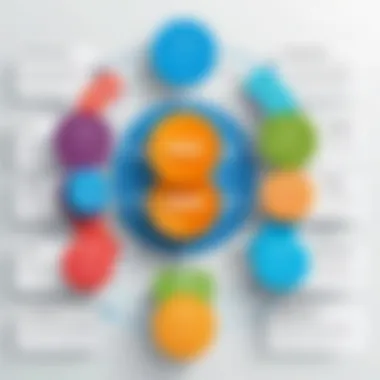
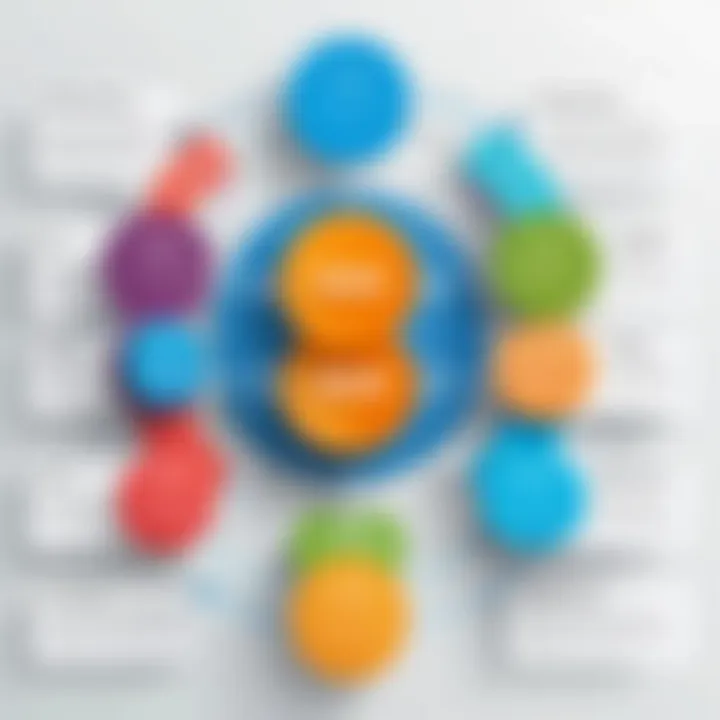
The significance of delineating the key steps in Master Data Management (MDM) cannot be overstated. MDM isn't a one-size-fits-all solution; rather, itâs a multifaceted effort that demands an intricate understanding of its various phases. By mapping out these essential steps, organizations can systematically enhance their data handling capabilities, ensuring data integrity, consistency, and accessibility across all departments. Each stage acts as a building block, facilitating smoother transitions to the next, and ultimately driving organizational efficiency.
Step One: Requirement Analysis
Gathering Stakeholder Needs
To kick off the MDM journey, as the name suggests, itâs crucial to get a handle on what stakeholders truly need. This isnât just about ticking boxes or nodding in agreement; itâs about actively listening to their pain points and aspirations. What challenges do they face with current data processes? Their insights provide a roadmap for what MDM should achieve. The key characteristic here is collaborationâbringing different voices to the table enables a rich tapestry of perspectives. However, if these views clash, it could lead to a convoluted direction for the MDM plan, making this step both vital and complex.
Understanding Data Sources
Next up is understanding the data sources at play. This is the backbone of any MDM initiative. Identifying where your data resides, whether in CRM systems like Salesforce or ERP platforms such as SAP, is essential. Each source may have different data quality and reliability levels, which can complicate the integration. The idea here is to evaluate the potential of each source, choosing those that align best with your organizational goals. A clear mapping of these sources can illustrate where redundancies or gaps exist, enhancing clarity and focus on what truly matters.
Defining Success Metrics
Itâs tough to measure success without a clear idea of what that looks like. This is where defining success metrics becomes paramount. These metrics will ultimately help assess the MDM initiativeâs impact, serving as benchmarks for performance evaluation. The critical characteristic of this step is specificityâbroad, vague metrics won't provide the actionable insights needed later on. They should relate directly to stakeholder needs and business objectives. However, crafting these metrics can be time-consuming, requiring careful thought to ensure accuracy and relevance.
Step Two: Data Governance Framework
Establishing Data Quality Standards
Once the requirements are analyzed, itâs time to set the stage for quality through establishing data quality standards. This involves defining what âhigh-qualityâ data looks like for your organization. Itâs a balancing act, as the standards must be achievable yet robust enough to maintain data integrity. Why is this important? Well, poor data quality can lead to misguided business decisions, ultimately affecting the bottom line. Establishing these standards also provides a framework for future data management efforts, creating a reference point for continuous improvement.
Defining Roles and Responsibilities
A robust data governance structure hinges on clearly defined roles and responsibilities. Who's in charge of what? This clarity is essential for accountability. Without it, chaos may ensue, and data management efforts could flounder. Itâs crucial for organizations to set up a hierarchy that delineates the tasks of various team members, from data stewards to IT professionals. This not only enhances workflow efficiency but also helps in managing stakeholder expectations, ensuring everyone knows their place in the MDM puzzle.
Implementing Data Stewardship
Data stewardship is where the rubber meets the road. Implementing effective stewardship roles ensures that thereâs someone âminding the storeâ when it comes to data quality. Stewards ensure that data is accurate, consistent, and used ethically throughout the organization. The unique feature of this approach is that it encourages a culture of accountability concerning data among all employees. While beneficial, it also requires investing time in training and promoting a shared understanding of data importance across teams.
Step Three: Data Model Design
Mapping Existing Data Structures
Data model design begins with mapping existing data structures. This involves taking stock of what is currently available and seeing how it fits into a larger schema. Understanding how data interacts with one another is vital for spotting inconsistencies and redundancies. This mapping process foregrounds the interconnectedness of various data sets, marking it as an indispensable choice for this article. However, if neglected, organizations might risk introducing new complexity or obscuring valuable data relationships.
Creating a Unified Data Model
The importance of creating a unified data model cannot be emphasized enough. This model acts as a centralized hub for data, ensuring that it is organized and easily accessible. A strong unified model will help in filtering out noise, allowing users to focus only on relevant data. Itâs imperative that this model is flexible enough to adapt as the organization evolves. On the flip side, overcomplicating it can deter effective usage, making proper design essential.
Aligning with Business Processes
Finally, aligning the data model with existing business processes completes the design stage. Without this alignment, data initiatives risk falling flat, disconnected from actual business needs. This characteristic is all about serving practical outcomes. When data management aligns seamlessly with processes, it becomes a powerful tool for success rather than a cumbersome hindrance. The danger lies in overlooking internal workflows, which can create mismatches in expectations and outcomes.
Step Four: Data Integration Strategy
Selecting Integration Tools
The selection of integration tools is a step that can truly make or break an MDM initiative. The right tools must synchronize data between various systems, ensuring a smooth flow of information. They should also be scalable to accommodate future growth. However, while exploring options, organizations might face challenges in evaluating compatibility with existing systems. Itâs essential to do a thorough examination, making informed choices that suit long-term needs.
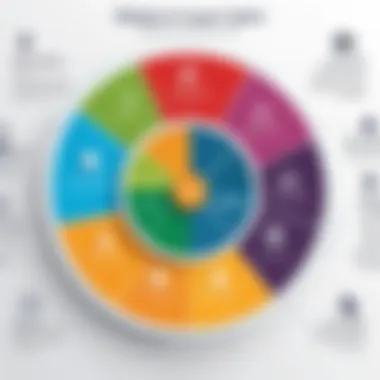
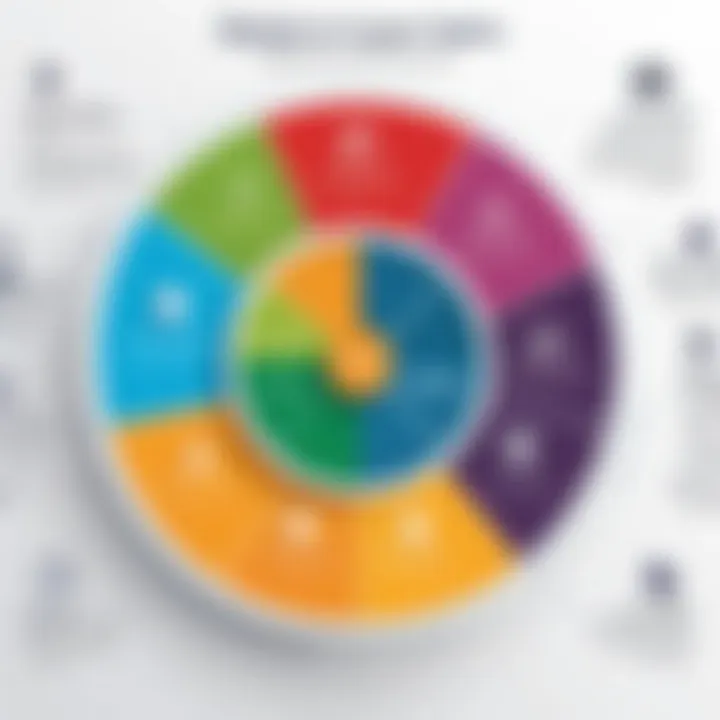
Data Migration Approaches
When it comes to data migration, organizations have different routes to consider. A comprehensive strategy may involve either a big bang approach or a phased one, each with its own pros and cons. A big bang approach can go live quickly but may introduce significant risk if something falters along the way. On the other hand, a phased approach allows for gradual adjustment but can stretch timelines. The key is to weigh these options carefully against organizational capabilities and readiness.
Real-Time vs Batch Integration
Deciding between real-time and batch integration ties closely to how swiftly organizations need their data available. Real-time integration offers immediate updates, essential for environments where timing is a critical factor, like financial transactions. Meanwhile, batch integration might work fine for less urgent applications, which can save resources. Understanding the business context is crucial to find the right fit, as choosing wrongly can lead to operational hiccups.
Step Five: Implementation and Deployment
Pilot Testing Solutions
Pilot testing MDM solutions is an indispensable phase that allows organizations to evaluate scalability and user experience before a full rollout. Running a pilot can reveal potential issues early on, saving resources down the line. However, the catch is that it requires adequate planning and investment upfrontâboth of which are critical for effective implementation. When done well, pilot testing can pave the way for smoother transitions and higher user buy-in in the long term.
Full Scale Implementation Strategies
Full scale implementation strategies need to be crafted with care. This phase includes not just deploying solutions but also ensuring that all stakeholders are informed and prepared. A unique element here is the necessity for strong communication plans to guarantee that users understand the new systems and processes. Nonetheless, rushing this phase could result in resistance from staff or failure to fully utilize the new tools.
Monitoring and Evaluation Processes
Monitoring and evaluation processes are crucial for continued success. These evaluations should align with the success metrics defined earlier, allowing organizations to gauge how well MDM initiatives are performing. By continually assessing performance, organizations can adapt and refine strategies to meet emerging challenges. However, failing to commit to ongoing evaluations may lead to stagnation, undermining the benefits achieved thus far.
Step Six: Maintenance and Evolution
Ensuring Data Quality Over Time
Ensuring data quality over time is a long-term commitment that shouldnât be overlooked. MDM is not a set-it-and-forget-it deal; ongoing audits and checks are necessary to uphold established data quality standards. The remarkable aspect of this effort is its ability to create a culture of data mindfulness across the organization, where everyone understands the importance of maintaining accuracy. On the downside, if ignored, data integrity can erode, rendering previous efforts moot.
Regular Updates and Reviews
Regular updates and reviews create an opportunity for continuous enhancement in the MDM framework. These check-ins allow organizations to adapt to new trends and technologies, preventing stagnation. It instills a proactive approach rather than a reactive one. However, this process can become burdensome without adequate resources, but the long-term benefits far outweigh the challenges if executed properly.
Adapting to Changing Business Needs
Finally, adapting to changing business needs is what keeps the MDM strategy relevant. Markets evolve, and businesses must pivot to meet new demands. This characteristic makes it essential for organizations to review their data strategies periodically. While the challenge lies in identifying shifts accurately and timely, organizations that successfully adapt position themselves better for optimization and growth.
Analyzing Success Factors
When it comes to Master Data Management (MDM), several key elements determine whether a project will thrive or merely survive. Analyzing the success factors isnât just a good idea; itâs critical for organizations wanting to get the most out of their data. In this section, weâll break down the importance of leadership support, user training, technology alignment, and continuous improvement practices. By understanding these fundamentals, businesses can create a well-rounded approach to MDM that not only kicks off successfully but also maintains its momentum over time.
Leadership Support and Commitment
Leadership plays a pivotal role in any MDM initiative. Itâs like the wind in a shipâs sails; without it, the journey can stall before it begins. Support from the top brass drives organizational commitment, ensuring that everyone understands the importance of master data. When leaders actively promote MDM, they help to facilitate resource allocation, prioritize tasks, and rally the team towards a common goal.
Moreover, leadership can model the right behaviors needed for effective data governance. When executives take an interest in MDM, it sends a strong signal to employees that this is not just another initiative to add to the list but rather a fundamental shift in how the organization treats data. This commitment can translate into enhanced accountability and participation across departments, paving the road for a successful MDM endeavor.
User Training and Adoption Strategies
No matter how sophisticated the systems are, without users who know how to leverage them, itâs a recipe for failure. User training isnât just about how to click buttons; itâs about instilling a data-centric mindset within the organization. Tailoring training programs to different user groups makes sure that everyone from data stewards to front-line employees understands their role and responsibilities.
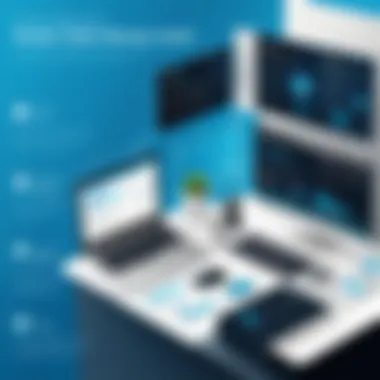
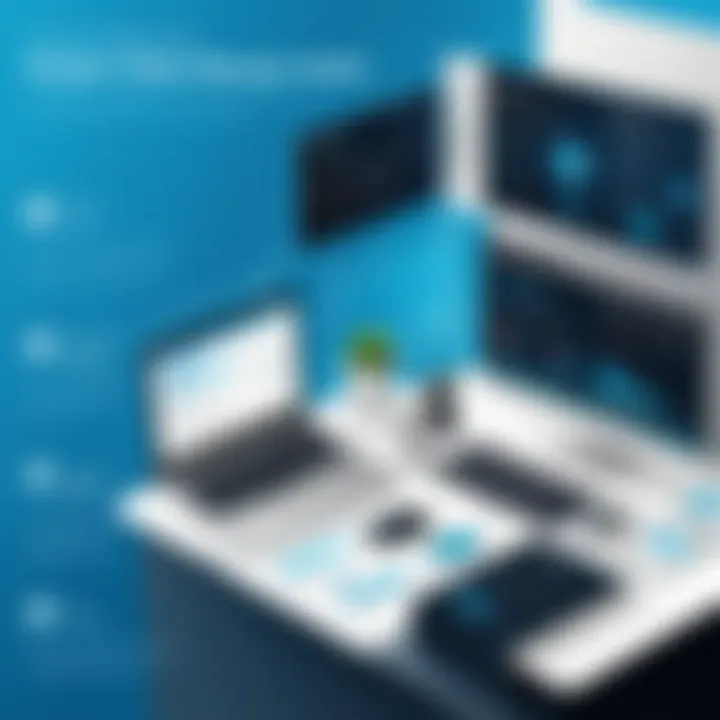
Adoption strategies should be part and parcel of the training efforts. These strategies can include hands-on workshops, e-learning modules, and even mentorship programs. Organizations can embrace the philosophy that âyouâre only as good as your dataâ. So, by investing in training, theyâd not only facilitate operational efficiencies but also contribute to overall business goals.
Technology Alignment and Tools
In the modern landscape, aligning the technologies used for MDM with organizational needs is as crucial as fitting a key into a lock. Tech misalignment can result in wasted resources, operational bottlenecks, and a marked resistance to MDM adoption. Businesses should assess their existing technologies and identify gaps that hinder effective data management.
Tools such as Informatica MDM, IBM InfoSphere, and SAP Master Data Governance can offer seamless integration with existing infrastructure. Selecting the right tools based on an organizationâs specific requirements can simplify the process of gathering, maintaining, and disseminating master data effectively. With the right technology in place, companies can enable quicker decision-making and greater agility in their operations.
Continuous Improvement Practices
MDM isnât just a one-and-done project; it requires ongoing efforts to adapt and evolve. Continuous improvement practices allow organizations to refine their MDM processes over time. Utilizing frameworks like Plan-Do-Check-Act (PDCA) ensures that teams regularly assess their performance metrics, identifying areas where adjustments are necessary.
Regular reviews can also help in staying relevant to industry standards and technological advancements. Organizations should cultivate a culture that welcomes feedback and change. This way, teams wonât be stuck in the past but can pivot quickly as new challenges arise. By creating a loop of continuous improvement, master data remains accurate, reliable, and above all, useful.
"The greatest danger in times of turbulence is not the turbulence; it is to act with yesterday's logic." - Peter Drucker
By focusing on these vital success factors, organizations can greatly enhance their chances of achieving a fruitful MDM implementation that supports their broader strategic goals and fuels data-driven decision-making.
Future Trends in Master Data Management
As we look ahead, the landscape of Master Data Management (MDM) continues to evolve, influenced by technological advancements and changing business priorities. Staying on top of these trends is crucial for organizations aiming to leverage data for competitive advantage. This section delves into some pivotal developments in MDM, emphasizing their importance and practical implications for todayâs businesses.
The Role of Artificial Intelligence
Artificial Intelligence (AI) is revolutionizing how organizations handle master data. By integrating AI into MDM processes, companies can automate data cleansing, classification, and enrichment. This not only increases efficiency but also enhances data accuracy. For instance, using machine learning algorithms, businesses can identify patterns and anomalies in data more easily, which leads to better decision-making.
Moreover, AI-driven tools can adapt and evolve, learning from new data inputs and user interactions. As a result, organizations can maintain high data quality and minimize human error. The ability for AI systems to analyze vast amounts of data at lightning speed is a game changer, allowing organizations to respond to market changes more swiftly.
"The integration of AI in MDM is akin to having a sharp scalpel in a surgeon's hand, enabling precision and minimizing the risk of errors."
Emerging Technologies and
The advent of emerging technologies such as blockchain, the Internet of Things (IoT), and advanced analytics are reshaping the MDM sphere in remarkable ways. For example, blockchain enhances data integrity by providing a decentralized and immutable record of data transactions. This is particularly beneficial in industries like finance and healthcare, where data trustworthiness is paramount.
The IoT contributes by generating an unprecedented volume of data from numerous devices, necessitating agile MDM solutions. Organizations can utilize this data for real-time analytics, which can inform operational strategies and customer engagement practices. Advanced analytics tools are becoming indispensable for deriving insights from vast data sets; thus, businesses can tailor their services to better meet client demands.
As these technologies continue to develop, their integration with MDM practices will enable organizations to unlock new opportunities, achieve greater efficiency, and enhance consumer experiences.
Increased Focus on Data Privacy
With regulations such as GDPR and CCPA making waves globally, the focus on data privacy within MDM strategies is more critical than ever. Organizations must navigate the complexities of data usage while simultaneously ensuring compliance with legal frameworks. This trend signals a shift from merely collecting and managing data to a more responsible and ethical approach to data stewardship.
Implementing robust data governance frameworks, educating employees on data privacy, and leveraging privacy-centric tools will become essential. Companies are beginning to see data privacy not just as a compliance necessity but as a strategic asset that can enhance their reputation and foster trust among customers.
The End: The Importance of in Data-Driven Decision Making
In today's fast-paced and data-driven world, the significance of Master Data Management (MDM) cannot be overstated. Organizations, whether small businesses or large enterprises, are inundated with massive amounts of data from various sources. MDM serves as the backbone that supports and structures this data, allowing companies to harness its full potential. Without a solid MDM strategy, data can become fragmented, inconsistent, and ultimately, unreliable. This fragmentation leads to poor decision-makingâa scenario no organization wants to find itself in.
MDM's importance is particularly highlighted in the following areas:
- Data Consistency: By consolidating data from multiple sources into a single, accurate version of the truth, MDM ensures that everyone in the organization is working from the same data set. This minimizes confusion and enhances trust in the data, which is critical for making informed decisions.
- Enhanced Analytics: With clean, consistent, and accessible data, organizations can dive deeper into data analytics. Effective data-driven decision making requires reliable data. MDM provides the quality data necessary for advanced analytics, enabling companies to uncover insights that might otherwise remain hidden.
- Regulatory Compliance: In an era of stringent regulations regarding data privacy and security, a well-defined MDM strategy aids in compliance efforts. Keeping data organized and tracked helps organizations quickly respond to regulatory audits, thereby avoiding penalties.
"Effective MDM is a journey, not a destination. Organizations that prioritize it can vastly improve their operational efficiencies and decision-making capacity."
- Operational Efficiency: Good Master Data Management streamlines operations within an organization. Redundant data processes can be eliminated, leading to a reduction in operational costs. It also enables employees to focus on value-added tasks rather than data entry and correction activities.
- Customer Satisfaction: A robust MDM approach helps maintain accurate customer records. This leads to a better understanding of customer needs and preferences, resulting in enhanced customer service. When customers feel valued and understood, their loyalty often increases.
In sum, MDM is more than just a technical implementation; it is an organizational mindset. The journey towards powerful data management requires a cultural shift that prioritizes the quality and integrity of data throughout the business. As organizations continue to evolve and face new challenges in a data-centric world, emphasizing MDM can lead to smarter, more agile decision-making processes. Thus, an effective MDM strategy is not just an optionâit's essential for those who want to thrive in competitive landscapes.