Understanding Big Data Analytics: A Comprehensive Overview
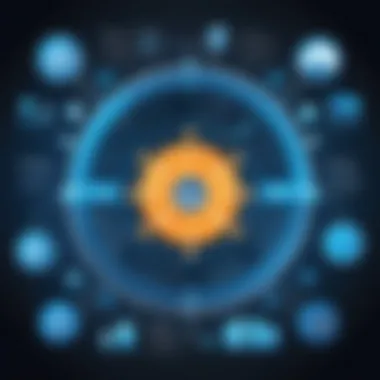
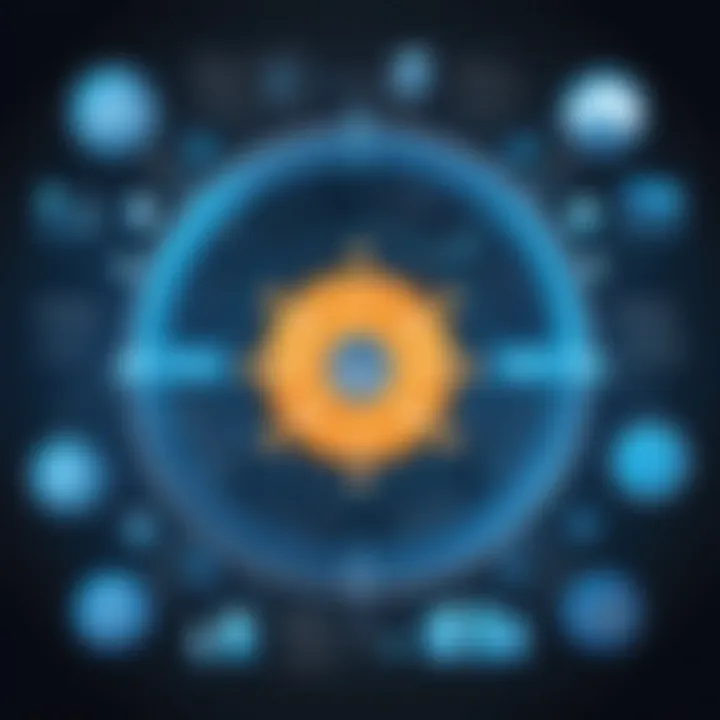
Intro
Big data analytics is not just a buzzword tossed around in the halls of corporate strategy meetings; it’s a vital tool that shapes how organizations operate at every level today. As the saying goes, “data is the new oil,” and similarly to oil, it requires the right refining processes to turn raw data into something truly valuable. This article peels back the layers on big data analytics, shedding light on what it really means, the techniques used, and how businesses can harness it for better decision-making.
In today’s digital landscape, businesses generate mountains of data daily—from customer interactions to operational processes. The trick lies in understanding how to interpret this data. Big data analytics includes a suite of techniques and tools that can make sense of this complexity. Companies across sectors, from healthcare to finance, have started to realize that insights drawn from data can be a game-changer. It can not only inform strategy but also predict trends, enhance customer experiences, and improve operational efficiency.
However, amid the potential lies a labyrinth of challenges that professionals encounter. Handling issues like data privacy, data integration, and ensuring data quality can present hurdles. This article aims to demystify these challenges while considering future opportunities and directions.
Let’s break down this nuanced topic into digestible sections, making it easier for IT and software professionals, as well as business leaders, to gather important insights and apply them effectively in their scenarios.
Software Overview
Software Description
In the realm of big data analytics, there’s a variety of software solutions designed to help organizations harness the power of their data effectively. These tools are pivotal in analyzing vast amounts of information, drawing out patterns, and providing actionable insights. Examples include SAS Analytics, Apache Hadoop, and Microsoft Power BI. Each software offers unique capabilities tailored to meet specific business needs.
Key Features
When considering effective big data analytics software, there are a few standout features to keep in mind:
- Data Integration: The ability to combine data from various sources, whether on-premises or in the cloud. This is often vital for establishing a holistic view of business operations.
- Real-time Analytics: Quickly processing data as it is generated, allowing businesses to respond swiftly to changing conditions.
- Visualization Tools: Graphical representations that help professionals dive into data findings without getting lost in a sea of numbers.
- Machine Learning Capabilities: Implementing algorithms that improve as they process more data, bringing predictive insights into play.
- User-friendliness: A designed interface that helps users, even those without a technical background, to utilize the software effectively.
With these features, organizations can not only analyze their data but also turn it into a strategic asset that drives growth and innovation.
User Experience
User Interface and Design
A user-friendly interface is fundamental for any software environment; even the most powerful analytics tools become ineffective if users can't navigate them efficiently. The best platforms tend to emphasize simplicity, easing the learning curve for users across various skill levels. Users should be able to find their way around, access critical features, and interpret data visualizations without spending an eternity figuring things out.
Performance and Reliability
The performance of big data analytics tools is another critical aspect. Software must handle massive volumes of data smoothly and without significant latency. A hiccup here can lead to delays in decision-making, which businesses can ill afford. Ensuring reliability means selecting tools that not only boast speed but are also scalable, so they can grow alongside the business.
"In the data age, the ability to reliably analyze data correlates directly with competitive advantage."
Understanding how each software handles performance under load and its consistency during peak usage is therefore vital for organizations eager to leverage data insights.
Collectively, these features and experiences highlight that understanding big data analytics is not a solitary journey. Rather, it’s a multifaceted landscape that needs careful navigation, deep understanding, and strategic implementation for success.
As we dive deeper into this narrative, we’ll explore foundational concepts, the challenges faced in the industry, and the far-reaching applications across different sectors, ultimately laying out a comprehensive view of this vital topic.
Prelude to Big Data Analytics
Big data analytics stands as a crucial focal point of conversation in today’s data-driven world. This section will lay the groundwork for understanding the significance of big data analytics, equipping readers with the knowledge to navigate the complexities surrounding this transformative field. As organizations begin to realize the vast potential hidden within data, the demand for acumen in big data analytics surges.
Grasping the nuances of big data analytics can provide businesses with invaluable insights that drive strategic decision-making. From enhanced customer experiences to optimizing operational efficiencies, the benefits extend across various industries. Understanding how to interpret and act on data insights can mean the difference between leading the pack or merely keeping up.
Defining Big Data
Big data refers to the colossal volumes of structured and unstructured data that inundate businesses daily. Yet, it’s not merely the scale that makes it significant; it’s how organizations make sense of this data. Traditional data management tools often falter when faced with such overwhelming quantities. Big data encompasses three main dimensions:
- Volume: Refers to the vast amounts of data generated every second. Think of social media interactions, purchase transactions, and sensor data from devices – it all adds up quickly.
- Velocity: Describes the speed at which data is created and processed. In the age of instant gratification, being able to analyze real-time data is crucial. Examples include live sports statistics and financial market changes.
- Variety: Indicates the different types of data. Whether it’s textual data from emails, audio files from calls, or video content from security cameras, variety is key to comprehensive analysis.
These facets together create a rich tapestry of information that, if harnessed properly, can yield powerful insights.
Understanding Analytics
Analytics itself is the science of analyzing raw data to uncover trends and patterns. Unlike simple data reporting, analytics employs sophisticated methods to extract meaningful information. This provides a clearer picture of past trends, current performance, and future forecasts.
Here are some key aspects of analytics:
- Descriptive Analytics: Focuses on summarizing historical data to understand what has happened. This might include generating reports on last quarter’s sales.
- Predictive Analytics: Uses statistical models and machine learning techniques to predict future outcomes based on historical data. A practical example would be forecasting sales based on seasonal trends.
- Prescriptive Analytics: Goes a step further by suggesting actions to benefit from potential future scenarios. Think of scenarios in supply chain management where analytics can recommend optimal inventory levels based on predicted demand.

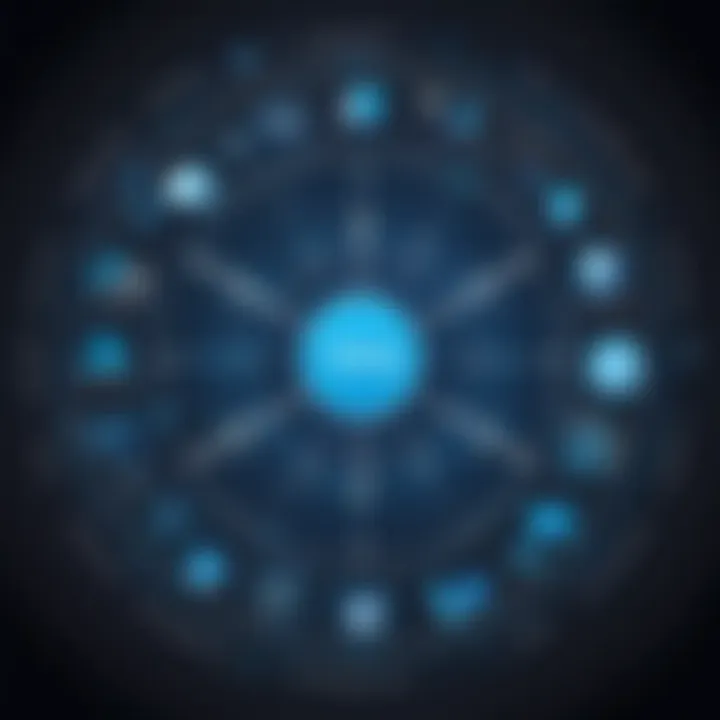
Understanding these different layers of analytics not only informs better decisions but also allows organizations to pivot quickly in response to market changes. In sum, the realms of big data and analytics are intertwined—a synergy that can propel organizations toward success when leveraged effectively.
The Intersection of Big Data and Analytics
The intersection of big data and analytics plays a pivotal role in understanding how organizations can unlock the wealth of information they possess. With businesses generating large volumes of structured and unstructured data, it becomes increasingly vital to harness analytics to derive actionable insights. This synergy between big data and analytics is not mere coincidence; it is a necessity in today’s data-driven landscape.
When we talk about big data, we’re looking at vast sets of information that cannot easily be processed using traditional methods. This includes data generated from various sources—websites, social networks, IoT devices, and transactions. Therefore, analytics steps in as the critical toolkit that enables businesses to sift through this ocean of data. By doing so, organizations can spot patterns, understand trends, and ultimately make informed decisions that drive success. In a sense, analytics acts like a compass in the unpredictable journey of big data.
Some specific benefits of merging big data with analytics include:
- Enhanced Decision-Making: With analytics, organizations can make decisions based on actual data rather than gut feelings or educated guesses.
- Personalization of Services: Businesses can tailor their offerings to better meet the specific needs and preferences of customers, creating a more engaging experience.
- Operational Efficiency: By analyzing processes, companies can streamline operations, minimize costs, and enhance productivity.
However, there are considerations to keep in mind. Not all data is created equal. Data quality is paramount; dirty, incomplete, or inconsistent data can lead to flawed analysis and misguided decisions. Moreover, organizations must navigate the challenges of integrating data from various sources, which often have diverse structures and formats. Therefore, strategic planning and the right technological tools are essential to ensure a seamless merger of big data and analytics.
The effective combination of big data analytics allows organizations to not only understand their current position but also anticipate future needs and challenges through predictive insights.
How Big Data Fuels Analytics
Big data fuels analytics through sheer volume and variety of data that can be analyzed. Traditional analytics techniques often fall short when faced with the complexities of big data. However, new technologies and methodologies are emerging to handle vast datasets effectively.
For instance, consider a retail company tracking millions of transactions daily. These datasets include purchase histories, customer feedback, social media interactions, and more. When analyzed using tools such as machine learning algorithms, the organization can uncover valuable purchasing patterns and seasonal trends—insights that were previously obscured due to data volume.
A few methods utilized for processing big data include:
- MapReduce: A programming model that processes large data sets by breaking them down into smaller, manageable parts.
- Data warehousing solutions: Such as Amazon Redshift or Google BigQuery, which help aggregate and analyze large quantities of data in one place.
- Real-time analytics platforms like Apache Kafka that can process streams of data as they arrive, enabling quick actions based on immediate insights.
The end result is a transformative understanding of consumer behavior and business operations—one that not only enhances efficiency but also boosts competitive advantage.
The Role of Data in Driving Insights
Data is the heart of analytics, serving as the foundational building blocks for insights. Without data, analytics would be akin to a ship lost at sea; it simply cannot navigate. Every data point, whether it's a number, a string, or a timestamp, contains potential insights waiting to be discovered.
In a way, data acts like a jigsaw puzzle. When assembled correctly, it paints a complete picture. Organizations need to gather data from various touchpoints to build this picture. Here are a few roles data plays in driving insights:
- Informs Strategy: Data-driven strategies allow for more accurate forecasts and stakeholder expectations by revealing key performance indicators (KPIs).
- Encourages Innovation: Insights derived from data analysis often lead to innovative product developments and improved services aligned with user needs.
- Improves Customer Relationships: Analytics help identify customer pain points, allowing businesses to address these challenges, thus strengthening loyalty.
Understanding how data can drive insights helps companies move beyond mere reactive measures. With a keen eye on data trends, organizations can proactively engage their audience, pivot during market shifts, and ultimately stay ahead of the competition.
Key Components of Big Data Analytics
Understanding big data analytics involves delving into several essential components that make it work effectively. These components play a crucial role in extracting actionable insights from vast datasets, guiding businesses and organizations in their decision-making processes. With the sheer volume, variety, and velocity of data being generated today, having a firm grasp on these elements can provide a competitive edge.
Data Sources
The backbone of big data analytics lies in data sources, which can originate from various places. They include but are not limited to social media interactions, transaction records, sensor data from IoT devices, and even unstructured data like texts and images. These diverse sources converge to form a rich data ecosystem, critical for analytics.
For businesses, understanding which sources provide valuable data is fundamental. By identifying relevant data streams, organizations can target their analytics efforts more effectively. For example, a retail brand might consider customer feedback from social media as vital input, while a healthcare provider might focus on electronic health records. This prioritization directly influences the success of subsequent data processing and analysis tasks.
Data Processing Technologies
Big data analytics wouldn't be feasible without robust processing technologies. Two prominent frameworks that stand out are the Hadoop Ecosystem and the Spark Framework. Each serves unique purposes but ultimately complements the analytics process by ensuring efficiency and scalability.
Hadoop Ecosystem
The Hadoop Ecosystem is a suite of tools designed for distributed storage and processing of large datasets. Its primary characteristic is the ability to handle vast amounts of information across clusters of computers, which allows organizations to scale their data storage effortlessly. One of its key advantages is how it employs the MapReduce programming model, breaking down large datasets into manageable tasks that can be executed in parallel.
"Hadoop enables organizations to tackle massive datasets that would boggle smaller systems."
However, while versatile, Hadoop can be slower compared to real-time frameworks because of its batch processing nature. This can be a drawback for businesses needing immediate insights.
Spark Framework
On the flip side, the Spark Framework is designed for high-level data processing with speed at its core. It provides real-time processing capabilities due to its in-memory data storage, making it faster than Hadoop for executing tasks.
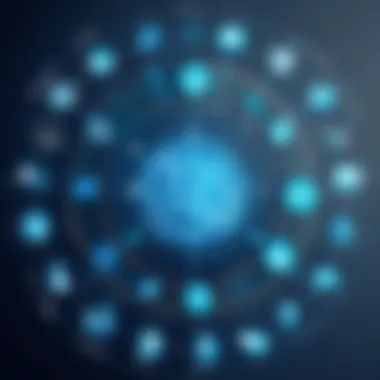
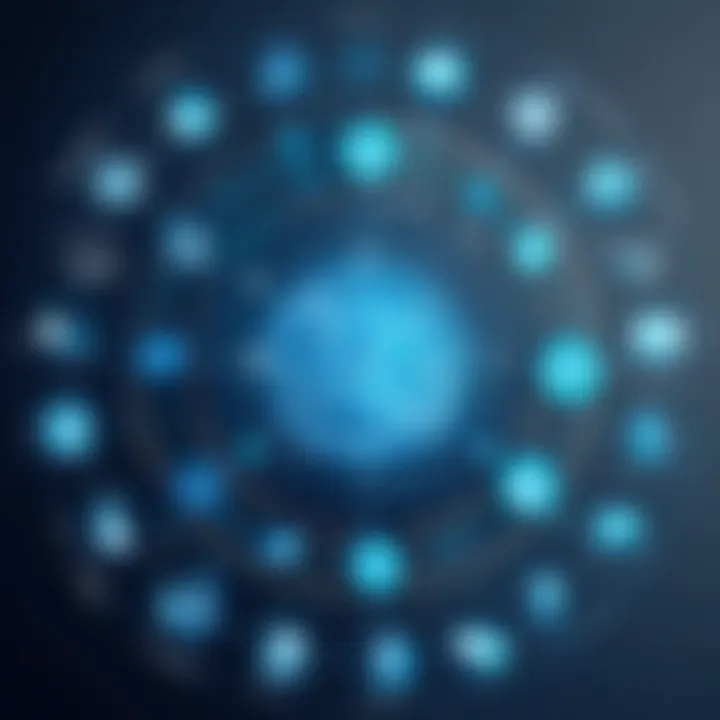
Spark's main benefit lies in its ability to process data at lightning speed, which is crucial for applications like fraud detection in financial transactions or real-time user personalization in eCommerce platforms. However, this speed comes with its own set of complexities when it comes to memory management and resource allocation, which could pose challenges for companies not equipped to handle them.
Analytics Techniques
To extract meaning from big data, various analytics techniques are employed. Each serves a different purpose, allowing organizations to draw insights tailored to their specific needs.
Descriptive Analytics
Descriptive Analytics provides a retrospective view of data, focusing on what has happened in the past. This form of analysis helps organizations understand historical trends and patterns. A key characteristic is its ability to present data in easily digestible formats, such as dashboards or reports.
While it's incredibly beneficial for reporting and tracking performance, descriptive analytics does have limitations. It primarily looks back in time, hence it cannot predict future outcomes or suggest courses of action, placing constraints on strategic planning.
Predictive Analytics
In contrast, Predictive Analytics goes a step further by utilizing statistical techniques and machine learning to forecast what might happen in the future. Its main appeal lies in its ability to identify correlations in large datasets and make predictions about future trends based on historical data. Companies across sectors—be it retail forecasting sales or healthcare predicting disease outbreaks—find value in its application.
However, implementing predictive analytics can be resource-intensive, requiring significant time and expertise to ensure accurate models.
Prescriptive Analytics
Lastly, we have Prescriptive Analytics, which not only anticipates what might happen but also suggests actionable steps to take. By leveraging algorithms and mathematical models, it can guide organizations on how to optimize operations and decision-making.
The unique aspect of prescriptive analytics is its decision-support capabilities; it can provide multiple scenarios and outcomes. However, the complexity involved in creating such models can deter organizations from adopting it fully, especially if they lack the technical know-how.
In summary, these key components of big data analytics form the foundation upon which companies can build their data strategies. Understanding these elements is essential for harnessing the power of big data effectively.
Applications of Big Data Analytics
The applications of big data analytics are practically limitless and span a multitude of industries. Leveraging vast amounts of data, organizations can identify trends, make informed decisions, and gain a competitive edge. Each sector utilizes big data analytics in unique ways to address specific challenges and enhance operational efficiency.
In Business Operations
Big data analytics is becoming the backbone of modern business operations. It aids in streamlining processes, optimizing supply chains, and improving overall productivity. Companies analyze consumer behavior, market trends, and internal performance metrics to make decisions based on real-time data rather than gut feelings. For instance, Amazon tailors recommendations to individual customers by analyzing browsing and buying patterns, significantly boosting sales.
Data-driven strategies allow businesses to allocate resources efficiently and respond swiftly to market changes. Organizations can evaluate performance across departments, identify bottlenecks, and adjust strategies to enhance profitability.
"Data is the new oil. It’s valuable, but if unrefined, it cannot really be used."
In Healthcare
The healthcare sector employs big data analytics to revolutionize patient care and operational efficiency. Hospitals and clinics collect and analyze data from patient records, clinical trials, and even real-time monitoring devices. For example, Mount Sinai Health System in New York utilizes predictive analytics to anticipate patient admissions, ensuring proper staffing and resources are available.
Moreover, big data analytics helps in analyzing the effectiveness of treatments, identifying outbreaks, and managing chronic diseases, thus enabling personalized medicine. It paves the way for better medical research by facilitating comprehensive data analysis on a large scale, ultimately leading to advancements in health outcomes.
In Finance
In finance, big data analytics is instrumental in risk management and fraud detection. Banks and financial institutions analyze transaction data in real-time to identify unusual patterns that may indicate fraudulent activity. Companies like Mastercard employ sophisticated algorithms to scrutinize millions of transactions every second, allowing them to mitigate risks efficiently.
Moreover, investment firms leverage big data to enhance trading strategies, evaluate market conditions, and forecast economic trends. By tapping into social media sentiments or transaction histories, they can make astute investment decisions, thus maximizing returns.
In Retail and eCommerce
Big data analytics in the retail and eCommerce sector helps businesses enhance customer experiences and optimize inventory management. Retail giants like Walmart analyze countless variables, from shopper behavior to seasonal trends, to refine their product offerings and marketers' strategies.
For eCommerce platforms, understanding customer interactions through clickstream data can lead to personalized shopping experiences that increase conversion rates. Retailers can use predictive models to manage stock levels and anticipate consumer demand, reducing both overstock and stockouts.
By applying big data analytics, retailers can not only maximize sales potential but also foster customer loyalty through tailored marketing approaches and relevant recommendations.
Challenges in Big Data Analytics
In the constantly evolving digital age, big data analytics stands as a double-edged sword. While it promises profound insights and unprecedented opportunities, the hurdles it presents are equally significant. Understanding these challenges is crucial, particularly for IT professionals and businesses that ride the waves of data. It’s not just about gathering vast amounts of data; it’s about making sense of it and ensuring its utility in decision-making.
Data Privacy Concerns
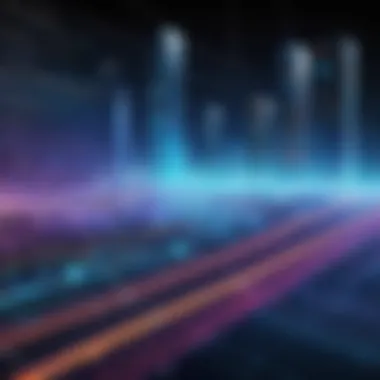
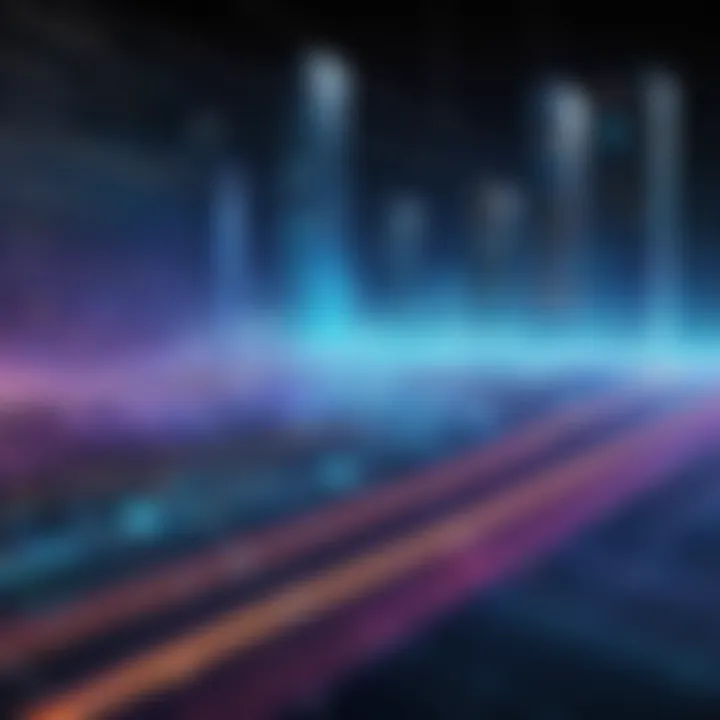
Data privacy is no longer a luxury; it's a necessity. With regulations like GDPR and CCPA taking center stage, companies must navigate a minefield of legal considerations. The data being analyzed often includes sensitive information, and mishandling it can lead to hefty fines and reputational damage. For example, if a health tech company inadvertently leaks patient data while analyzing trends in treatment effectiveness, the fallout could be catastrophic—not just financially but also in patient trust.
To maintain a balance between insightful analytics and privacy, businesses are opting for anonymization techniques and encryption. However, this can often alter the quality of the insights derived.
"Data is a new oil; it's valuable but harmful if leaked."
This statement emphasizes the need for strict compliance and proactive measures in data handling.
Data Quality and Integrity
Data is only as good as its integrity. If the data is erroneous, its analysis will yield misleading insights. Businesses often face issues where data from disparate sources does not correlate or is incomplete. For instance, if a retail business integrates its sales data from in-store transactions with online data but fails to account for returns or discounts accurately, the resulting analysis could paint an inaccurate picture of revenue trends.
Ensuring data quality often necessitates implementing rigorous validation processes and regular audits. Companies are investing in data governance frameworks, yet the practice can be labor-intensive and requires constant monitoring.
Steps to Improve Data Quality:
- Implement data validation rules.
- Regularly update databases to remove outdated or incorrect information.
- Involve stakeholders in data entry processes to ensure accuracy.
Scalability Issues
As businesses scale, the volume of data they generate swells dramatically. This growth can lead to significant scalability challenges. A small startup, once operating on a simple database, may find that as its customer base grows, the initial infrastructure becomes inadequate. Without the right frameworks in place, analytics can lag, becoming a bottleneck in the decision-making process.
To combat scalability woes, companies are looking at cloud-based solutions that offer flexibility and easier management. These platforms can accommodate fluctuations in data volume without necessitating massive investments in physical infrastructure. However, the need for skilled personnel to manage and optimize these solutions creates another layer of complexity.
In summary, while big data analytics fosters informed decision-making and growth, the challenges it presents are notable. By understanding and addressing data privacy, quality, and scalability concerns, businesses can harness the true power of data, turning potential pitfalls into stepping stones for success.
Future Trends in Big Data Analytics
As we navigate through the complexities of big data, it becomes increasingly clear that the landscape is ever-changing. The future trends in big data analytics not only highlight the advancements in technologies but also underline the changing dynamics of how businesses operate. By staying attuned to these trends, organizations can harness the potential of data, ensuring a competitive edge in their respective fields.
Artificial Intelligence and Machine Learning
Artificial Intelligence (AI) and Machine Learning (ML) are the game-changers in the realm of big data analytics. With the sheer volume and velocity of data generated daily, traditional data analysis methods struggle to keep up; that's where AI and ML step in. These technologies can autonomously analyze vast datasets, identifying patterns and insights that might easily go unnoticed by human analysts.
- Personalization: AI facilitates tailored experiences for customers by predicting their preferences based on previous behaviors. Businesses can enhance customer satisfaction and retention significantly this way.
- Predictive Maintenance: In sectors like manufacturing, AI can predict when a machine might fail, allowing preemptive action to be taken, thus minimizing downtimes.
- Cost Efficiency: Organizations can save money by reducing human error in data processing and analysis, leading to smarter budgeting and resource allocation.
One can't underestimate the impact of AI and ML in boosting decision-making speed and accuracy. With advancements in these technologies, the future holds promises of not just faster but smarter analytics.
Real-time Data Analytics
The ability to analyze data in real-time is rapidly shifting from a luxury to a necessity. As consumers crave instantaneous results and insights, businesses must adapt by incorporating real-time data analytics into their strategies. This capability allows organizations to respond quickly to market trends, customer behaviors, and operational challenges as they arise.
- Instant Feedback: Companies can gauge the effectiveness of marketing campaigns or product launches almost immediately, allowing fine-tuning in real time.
- Enhanced Customer Service: By analyzing customer interactions as they happen, businesses can address issues and queries on the fly, boosting customer satisfaction.
- Operational Efficiency: Real-time analytics helps in monitoring operations, ensuring that performance metrics are met consistently, thus reducing waste and increasing productivity.
Automation of real-time analytics processes will likely lead to greater accessibility, empowering small and mid-sized businesses to leverage these tools effectively.
The Growth of Edge Computing
Edge computing is garnering attention as a pivotal trend in the big data analytics ecosystem. As data is processed closer to where it is generated, rather than relying solely on centralized data centers, the advantages become evident.
- Reduced Latency: By processing data on-site, organizations can achieve lower latency, which is critical for applications that rely on immediate insights.
- Increased Security: Keeping data processing local can aid in reducing privacy risks, as less sensitive data is transmitted over networks.
- Bandwidth Efficiency: Edge computing alleviates the load on cloud infrastructures by minimizing the amount of data that needs to be communicated over the internet. This also translates to cost savings in bandwidth consumption.
The accelerating adoption of IoT devices opens up further possibilities for edge computing. Businesses can leverage real-time insights from data collected at the edge, fostering smarter decision-making and operations.
The End
In the realm of information and technology, the conclusion of an article on big data analytics acts as a mirror, reflecting the various components discussed throughout. It emphasizes the intricate tapestry woven with threads of data, analytics techniques, and real-world applications. No longer just a buzzword, big data analytics stands as a pivotal pillar in the operational strategies of organizations, bridging the gap between sheer data accumulation and actionable insights.
Summarizing the Importance of Big Data Analytics
Big data analytics holds substantial significance across various domains. To condense its importance into a few key elements:
- Informed Decision-Making: Organizations that utilize big data effectively can make more informed choices. For instance, a retail company analyzing customer purchase trends can optimize inventory levels, tailoring products to local demand, ultimately leading to increased sales.
- Competitive Advantage: The ability to harness data translates to a competitive edge. Companies levering big data analytics can outperform rivals by predicting market changes accurately. Consider the impact of Netflix predicting viewer preferences, enabling them to produce shows that capture audience interest.
- Enhanced Operational Efficiency: By understanding their processes through data analysis, businesses can streamline operations. For example, logistics firms can analyze delivery data to minimize routes, lowering costs and improving service reliability.
- Personalization: Customers now expect tailored experiences. Big data allows businesses to deliver personalized marketing and services. Think about how Spotify creates personalized playlists based on listening habits – a direct result of analyzing vast amounts of user data.
These core elements showcase how big data is not just a trend but a necessity for modern enterprises. As we further navigate the complexities of data, understanding its analytic potential will be crucial for sustained growth within any industry.
Final Thoughts on the Future Landscape
As we look ahead, the landscape of big data analytics is set to evolve in significant ways. Several considerations will shape its trajectory:
- Integration of AI and Machine Learning: The future will see deeper integration of artificial intelligence. As algorithms become more sophisticated, the capability to analyze unstructured data will enhance, enabling businesses to extract previously untapped insights.
- Real-Time Analytics: Streaming data is becoming the norm. Companies needing immediate insights for agile decision-making will lean heavily on real-time analytics solutions, refining how businesses react to market changes.
- Focus on Data Governance: With increased reliance on data comes the necessity for robust data governance practices. Organizations will need to prioritize protecting personal information and ensuring data integrity.
- Edge Computing: As more devices become connected, processing data closer to its source will become more vital. Edge computing allows for quicker analytics, which is critical for applications ranging from IoT to automated manufacturing.