Understanding SPSS: Key Features and Applications
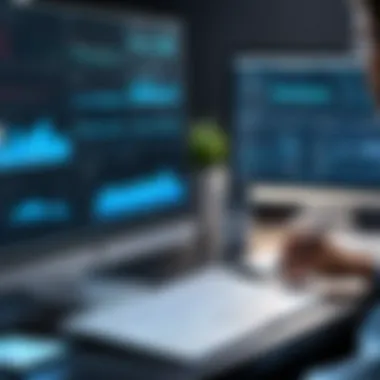
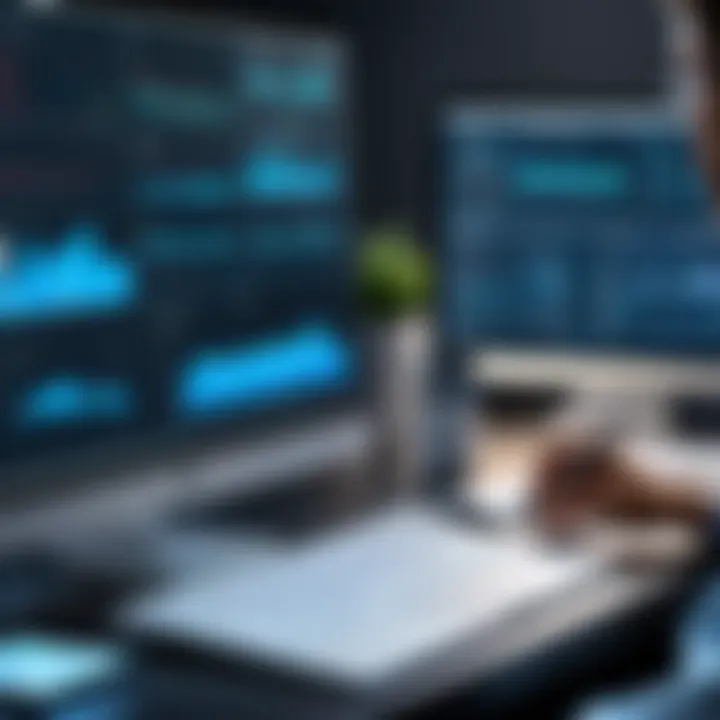
Intro
SPSS, or Statistical Package for the Social Sciences, has become a cornerstone in the realm of data analysis. It is widely used by researchers, educators, and businesses for its robust statistical capabilities. This section begins to outline its significance in various fields. From psychology to marketing analysis, SPSS provides effective tools for interpreting complex data.
In todayโs society where data-driven decisions shape the future, mastering SPSS is increasingly beneficial. This article will explore the software thoroughly, enabling users to unlock its full potential. Understanding its fundamental features, overall functionality, and practical applications can lead to better analytical outcomes.
Software Overview
Software Description
SPSS was originally introduced in 1968, evolving significantly over the decades. It is versatile for various statistical methods such as regression, ANOVA, and descriptive statistics. The software is designed to simplify complicated data sets, making them manageable.
Users can easily import data from multiple sources. These include CSV files, Excel sheets, or database systems. SPSS supports big data analytics, allowing it to handle extensive data sets effectively. Its focus on usability makes it a common choice among non-statisticians.
Key Features
SPSS offers several imperative features:
- User-friendly Interface: Even beginners find it intuitive.
- Interactive Graphs: Visual representation of data is straightforward yet powerful.
- Extensive Output Options: Flexibility in presenting data findings.
- Advanced Statistical Techniques: Options for complex analyses are plentiful.
SPSS aids in minimizing the time spent on data preparation, allowing more focus on interpretation and decision-making.
The software provides a wide array of statistical tests and modeling techniques. Users can run frequencies, explore crosstabs, or conduct more complex regression analyses in no time. The variety allows professionals from different industries to meet their specific analytical requirements.
User Experience
User Interface and Design
The user interface is crucial for efficiency, especially in analytical tasks. SPSS maintains a clean and organized layout. This design allows users to navigate through various features without confusion. Icons and drop-down menus facilitate quick access to functions, promoting a seamless workflow.
Furthermore, SPSS enables users to customize their workspace. Users can choose the arrangement of the tools they frequently use, streamlining their personal experience.
Performance and Reliability
In terms of performance, SPSS stands out for processing speed. Users have noted that the software efficiently handles large datasets without significant slowdown. Reliability is another key aspect; SPSS features rigorous testing and updates, ensuring that statistical calculations remain accurate and up-to-date.
The software can run on both Windows and Mac systems, making it accessible to a broader audience. Thus, it brings together a diverse user base spanning academia and various industries.
Prologue to SPSS
The importance of understanding SPSS cannot be overstated for those engaged in data analysis. This software tool serves as a crucial asset for professionals seeking to extract insights from complex data sets. By exploring SPSS, users gain valuable knowledge about data manipulation, statistical techniques, and the presentation of findings. Hence, a clear introduction to SPSS lays the groundwork for deeper exploration into its capabilities.
Overview of SPSS Software
SPSS, or Statistical Package for the Social Sciences, is a powerful statistical software tool used widely for data analysis. Its user-friendly interface and comprehensive features enable researchers to perform a variety of statistical tests, from basic descriptive statistics to complex multivariate analyses. Users can easily manage data files, manipulate data sets, and obtain output in an understandable format. This flexibility caters to a broad range of disciplines, including social sciences, business, and healthcare, making it an essential tool in the realm of data-driven decision-making.
Some key features of SPSS software include:
- Data Management: Allows users to import, export, and manage data with ease.
- Statistical Analysis: Supports both descriptive and inferential statistics.
- Graphical Tools: Provides options for data visualization, such as charts and plots, facilitating clearer communication of results.
As a comprehensive program, SPSS supports users in developing a thorough understanding of their data. It is not only beneficial for statistical novices but also for seasoned analysts looking for reliable outputs.
History and Evolution of SPSS
The history of SPSS traces back to 1968, when Norman H. Nie, William A. C. Hull, and William H. (Bill) Stein created the first version. Initially intended for social science research, SPSS quickly evolved, gaining traction in various fields due to its ability to process and analyze large data sets efficiently. Over the years, SPSS has adapted to advancements in technology.
As computers became more integral to research, SPSS incorporated new functionalities. By the 1980s, the software transitioned to personal computers, widening its user base. The introduction of point-and-click interfaces further simplified access for those unfamiliar with programming languages.
In time, significant updates included enhanced data management features, the development of syntax language for advanced users, and specialized modules for business and health analytics. In 2010, IBM acquired SPSS Inc., marking a significant milestone that aimed to integrate SPSS capabilities with IBM's analytics solutions. This evolution illustrates how SPSS continued to meet the changing needs of data analysts, becoming a staple across numerous industries.
"SPSS has evolved from a tool for social researchers into one of the most widely used data analysis software programs in the world."
The trajectory of SPSS underscores its pivotal role in fostering a data-centric culture, equipping users to make informed decisions based on their findings.
Key Features of SPSS
The key features of SPSS software serve as the foundation for its utility in various research and analysis contexts. These features not only enhance the capability of users to conduct complex analyses but also improve the efficiency of data handling. Understanding these features is crucial for anyone looking to leverage SPSS in their work.
Statistical Analysis Capabilities
SPSS is renowned for its powerful statistical analysis capabilities. It offers a broad range of statistical tests, making it a favorite among researchers across different fields. The software supports both descriptive and inferential statistics. Users can perform tasks ranging from simple means and frequencies to advanced regression analyses and multivariate testing.
One significant advantage of SPSS is its user-friendly interface, allowing even those without a strong statistical background to execute sophisticated analyses. The software includes built-in functions that facilitate various types of statistical procedures. For example, you can run t-tests, ANOVAs, and correlation analyses with minimal effort. Moreover, SPSS provides options to customize analyses, enabling users to tailor outputs to their specific needs.
Such flexibility in statistical analysis ensures that SPSS remains relevant across different research contexts, whether in social sciences, market research, or healthcare.
Data Management Functions

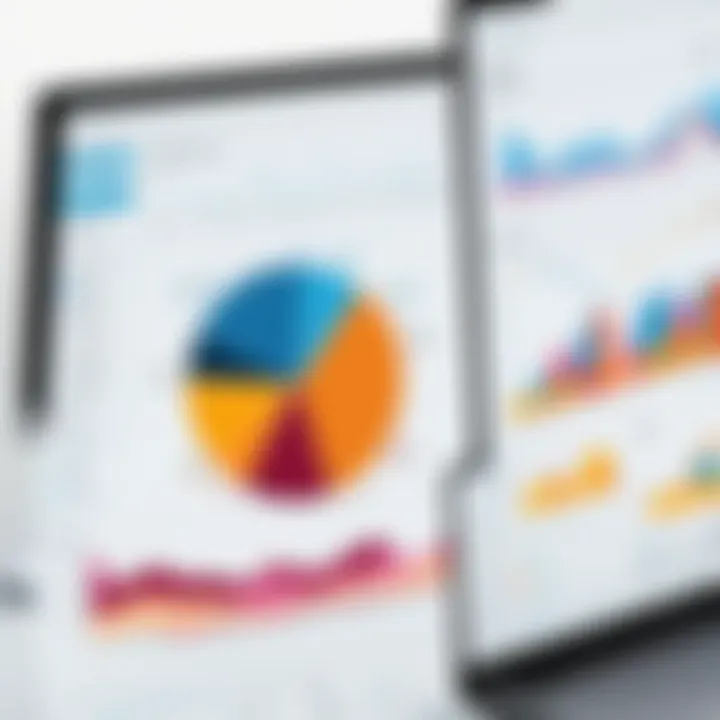
Effective data management is crucial in research, and SPSS excels in this area. One of the essential features is its data manipulation capacity. Users can easily import data from various formats such as Excel, CSV, or even databases. Once in the system, data can be managed efficiently, including sorting, filtering, and recoding variables.
Data cleaning is another vital function within SPSS. This ensures that the data set is free from errors and outliers, improving the quality of analyses. Users can conduct missing value analysis and replace or exclude missing data based on established criteria. Documentation is also facilitated, enabling researchers to keep track of changes made during the data management process.
Additionally, SPSS supports large data sets, which is beneficial for organizations that require heavy data lifting. This capability allows for comprehensive analysis even with extensive amounts of data.
Graphical Representation Tools
Visual representation of data is crucial for interpretation and presentation, and SPSS provides numerous tools for this purpose. Graphical options in SPSS include histograms, scatter plots, box plots, and bar charts. These features allow users to present their findings in an accessible way, making complex data more understandable to audiences.
Through the platform's customization options, users can modify graphs to align with specific presentation needs. This flexibility ensures that the graphical output not only conveys information effectively but also adheres to the branding and stylistic preferences of the user or organization.
Furthermore, the graphical capabilities of SPSS are complemented by its statistical analysis functions, allowing users to visually represent complex relationships and trends derived from their analyses. This integration enhances communication of results, making it easier to share insights with stakeholders, whether in reports or presentations.
Understanding the features of SPSS can significantly enhance your analytical capabilities, allowing for more informed decision-making processes in your organization.
Applications of SPSS in Research
The applications of SPSS in research are broad and significant. SPSS stands as a versatile tool that enables researchers to analyze data effectively across different domains. Understanding its applications helps in harnessing the full potential of this software. The effective use of SPSS can lead to more accurate insights, better decision-making, and overall enhanced research outcomes.
Social Science Research
In social science research, SPSS is invaluable. It assists researchers in conducting surveys, experiments, and observational studies. The software allows users to easily manage large datasets, which is often a challenge in social sciences. With SPSS, researchers can perform various statistical tests, such as t-tests, ANOVA, and chi-square tests. These statistical methods help in understanding relationships between variables, testing hypotheses, and uncovering social trends.
"Using SPSS enables social scientists to delve deep into human behaviors and societal patterns with precision."
Moreover, the graphical representation of data aids in presenting complex information clearly. Researchers can generate charts and graphs to illustrate their findings effectively, making it easier for audiences to digest the information. SPSS also provides functionalities for factor analysis, allowing researchers to identify underlying relationships between measured variables.
Market Research Analysis
Market research is another field where SPSS shines. Businesses rely on market research to understand consumer behavior, preferences, and market trends. SPSS enables market researchers to analyze survey results, sales data, and customer feedback accurately. Its data management capabilities simplify the handling of large sample sizes, which is essential in market studies.
Researchers can conduct segmentation analysis, helping businesses understand distinct consumer groups. This analysis is critical for developing targeted marketing strategies. Further, SPSS facilitates predictive modeling, where researchers can forecast trends and consumer behavior based on historical data. Such predictive insights are valuable for strategic planning and resource allocation.
Healthcare Data Analysis
In healthcare, the significance of SPSS cannot be overstated. This software aids in analyzing clinical trial data, patient surveys, and epidemiological studies. Healthcare analysts can utilize SPSS for descriptive analyses, such as mean comparisons, and also for complex inferential statistics, which are crucial in understanding health outcomes.
SPSS supports researchers in identifying factors that contribute to health issues or treatment outcomes through regression models. These analyses can lead to informed decisions in public health policy and clinical practices.
Furthermore, the software can manage missing data effectively, a common issue in healthcare research. By employing techniques like imputation, researchers can enhance the quality of their analyses, leading to more reliable results.
Installation and Setup
The significance of Installation and Setup cannot be understated when it comes to using SPSS effectively. Proper installation ensures that the software runs smoothly, and understanding the setup process prepares users for future operations. You need to consider your systemโs compatibility, configuration for optimal performance, and recognition of any potential issues prior to installation. This attention to detail saves time and reduces frustration later on in the data analysis process.
System Requirements for SPSS
Before installing SPSS, it is crucial to verify if your system meets the required specifications. SPSS runs on various operating systems, but each version may have distinct needs. Generally, here are the common requirements:
- Operating System: Windows 10 or higher; macOS 10.12 or higher
- Processor: Intel or AMD processor that supports 64-bit computing
- RAM: Minimum 4GB; recommended 8GB or more for heavy data processes
- Storage: At least 2GB of free disk space; SSD is preferable for enhanced speed
- Display: A minimum resolution of 1024 x 768 pixels
Performance tip: Ensure your system meets or exceeds these requirements for optimal operations.
Installation Process Guide
Once you've confirmed that your system is compatible, follow these steps for installation. SPSS installation is generally straightforward, and can be completed in a few steps:
- Download the Software: Retrieve the installation file from the official IBM SPSS website. Make sure you download the correct version for your operating system.
- Run the Installer: Locate the downloaded file, usually in your 'Downloads' folder. Double-click the file to initiate the installation process.
- Follow the Prompts: The installation wizard will provide guidance on each step. Review the license agreement, and select the installation type (Typical is recommended for most users).
- Enter License Information: You may need to input the license code provided at the time of purchase. This code validates your software use.
- Select Installation Directory: You can choose where SPSS will be installed, or simply use the default settings.
- Complete Installation: Click the install button and wait for the process to finish. It may take several minutes based on your system's speed.
- Restart Your System: Once the installation is complete, reboot your computer to apply the changes.
Important: Always err on the side of caution and ensure you have administrative rights on your machine to avoid errors during installation.
Following these steps will lead you smoothly into the realm of data analysis with SPSS, preparing you to explore its features and functionalities with confidence.
User Interface Overview
The user interface of SPSS is a crucial element for both novice and experienced users, as it plays a significant role in the overall effectiveness and efficiency of data analysis. A well-designed interface enhances user productivity and minimizes frustration, allowing individuals to focus on their analytical tasks rather than navigating complexities. Understanding this interface is also important to ensure better comprehension of SPSSโs capabilities and functionalities.
Navigating the Toolbar
The toolbar in SPSS is essentially the nerve center for users to access various functions and tools. It offers a range of icons and drop-down menus that facilitate quick actions, such as opening files, running analyses, or generating graphs. Each icon is designed to simplify the userโs journey through the software, making it essential to become familiar with these tools.
Key elements in the toolbar include:
- File Management: Options to create, open, and save datasets.
- Edit Features: Functions to cut, copy, and paste data, aiding in data manipulation.
- Analyze Menu: Direct access to the most commonly used statistical procedures.
Getting accustomed to the toolbar can significantly reduce the time spent searching for tools, allowing for a smoother workflow and more efficient analysis.
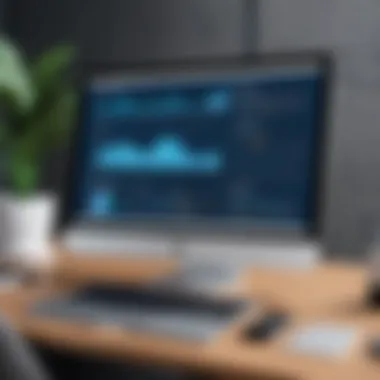
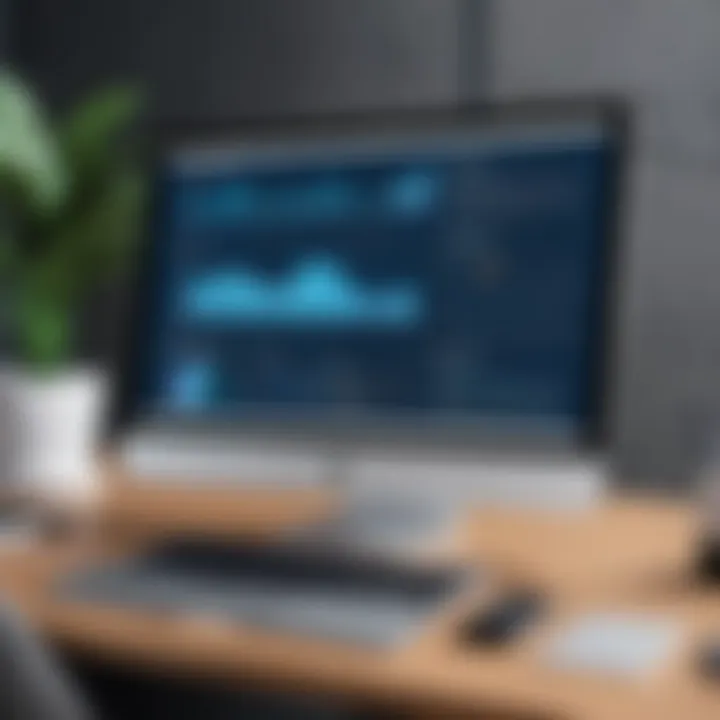
Understanding the Output Window
The output window in SPSS is where the results of your analyses are displayed. Understanding this window is vital because it presents raw outputs, tables, and graphical representations in a clear manner. Knowing how to interpret these outputs is essential for drawing meaningful conclusions from your data.
In the output window, you will typically see:
- Tables: Representing data summaries or statistical test results.
- Graphs: Visual representations that enhance understanding of data patterns.
- Logs: Information regarding the commands you executed, which can be invaluable for future reference.
Users should learn to navigate through the output efficiently. A well-organized output can help in identifying trends and relationships in the data, leading to better-informed decisions.
Using the Data Editor
The Data Editor is another key component of SPSSโs user interface. It functions as a spreadsheet-like view where users can input, view, and edit their datasets. A solid understanding of the Data Editor is essential because data preparation is a critical step in any analysis.
In the Data Editor, you can:
- Input Data: Enter data manually or paste it from other sources seamlessly.
- Modify Variables: Change variable names, types, and formats to suit your analysis requirements.
- Perform Basic Functions: Conduct basic computations, such as aggregation or reformatting data instantly.
Mastering the Data Editor allows for precise control over your datasets, which ultimately contributes to the quality of your analyses.
"Familiarity with SPSS's user interface is not just about usability; itโs a gateway to leveraging the powerful statistical capabilities of the software effectively."
Data Analysis Techniques
Data analysis techniques play a crucial role in transforming raw data into valuable insights. They provide methods for examining, processing, and interpreting data in a meaningful way. In the context of SPSS, these techniques empower users to uncover patterns, validate hypotheses, and make data-driven decisions. Understanding these techniques is invaluable for researchers and business analysts alike, as they can influence strategic initiatives and operational efficiencies.
Descriptive Statistics
Descriptive statistics summarize the main features of a dataset. These statistics provide an overview, allowing users to understand the data quickly. In SPSS, users can compute measures such as mean, median, mode, and standard deviation with ease. Simple yet powerful, descriptive statistics help in data exploration by presenting key information without detailing inferential associations.
Key outputs from descriptive statistics include:
- Measures of Central Tendency: These include the mean, median, and mode, which depict the central point of the data.
- Measures of Dispersion: These describe the spread of data points such as range, variance, and standard deviation.
- Frequency Distribution: It illustrates how often each value occurs within the dataset.
Utilizing these measures allows users to quickly gauge the data's behavior and identify anomalies or outliers in the dataset. Understanding how to implement descriptive statistics in SPSS is the first step towards mastering more complex analytical techniques.
Inferential Statistics
Inferential statistics enable users to make predictions or inferences about a population based on a sample subset. This can be particularly useful when examining whether findings from a small group apply to the larger population. SPSS offers a suite of tools for conducting various inferential statistical tests, including t-tests, chi-square tests, and ANOVA.
Key components of inferential statistics include:
- Hypothesis Testing: Used to determine the validity of assumptions regarding the data.
- Confidence Intervals: Provide a range of values where the true population parameter is expected to lie.
- P-values: Help in evaluating the significance of results in hypothesis testing.
Utilizing these techniques allows researchers to draw valid conclusions about broader trends, benefiting data-driven strategies in sectors such as social sciences, marketing, and healthcare.
Regression Analysis
Regression analysis serves as a powerful technique for modeling relationships between variables. It helps in predicting outcomes based on independent variables while analyzing their interaction. In SPSS, regression can take various forms such as linear regression, logistic regression, and multiple regression, depending on the nature of the data and the relationships being studied.
The primary focuses of regression analysis include:
- Model Building: Creating mathematical representations to illustrate the relationship between dependent and independent variables.
- Predictive Analytics: Informing future trends or values based on existing data patterns.
- Coefficient Interpretation: Understanding the influence each independent variable has on the dependent variable.
Regression analysis is critical for making informed predictions and understanding how different factors may affect outcomes. By mastering this technique, users can navigate more complex datasets and derive actionable insights efficiently.
"Effective data analysis techniques are essential in deriving meaningful results from complex datasets, enabling informed decision-making across various domains."
By understanding these essential data analysis techniques in SPSS, users can enhance the quality and effectiveness of their analytical projects.
Advanced SPSS Features
Advanced features of SPSS enhance the capability of users in executing complex data analysis tasks. Understanding these features is essential for those who seek to leverage the full potential of the software, especially in professional environments like research institutions, academic settings, and corporate data analysis units. The advanced functionalities not only save time but also improve accuracy and efficiency in data processing.
Syntax Editor Utilization
The Syntax Editor within SPSS is a powerful tool that allows users to write, edit, and execute syntax commands. This feature is particularly beneficial because it enables reproducibility of analyses. By saving commands as syntax files, you reduce the likelihood of user error that can occur with point-and-click operations. Instead of repeating procedures manually, users can execute batches of analysis with a single command.
Utilizing the Syntax Editor facilitates several benefits:
- Reproducibility: Documenting your analysis steps ensures that others can replicate your results.
- Efficiency: Running commands in bulk significantly speeds up the data analysis process.
- Customization: Users can create specific scripts tailored to their unique analytical needs, enabling more complex functionality.
To start using the Syntax Editor, simply navigate to the File menu, select New, and choose Syntax. Users can then write their commands in a straightforward, clear manner to maintain organization and clarity.
Customizing Outputs
Customizing outputs is another advanced feature that enhances user experience in SPSS. The default output format may not always meet specific needs, and being able to tailor outputs to particular requirements is valuable. Users can alter charts, tables, and various visual representations to better communicate results. For instance, modifying colors, fonts, and layout helps to create outputs that align with presentation standards or specific audience preferences.
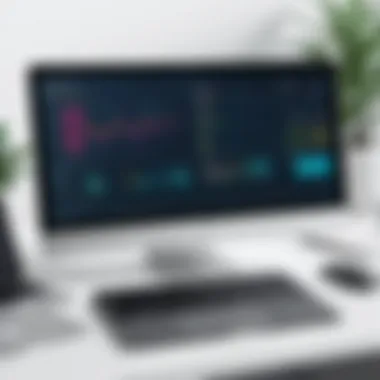
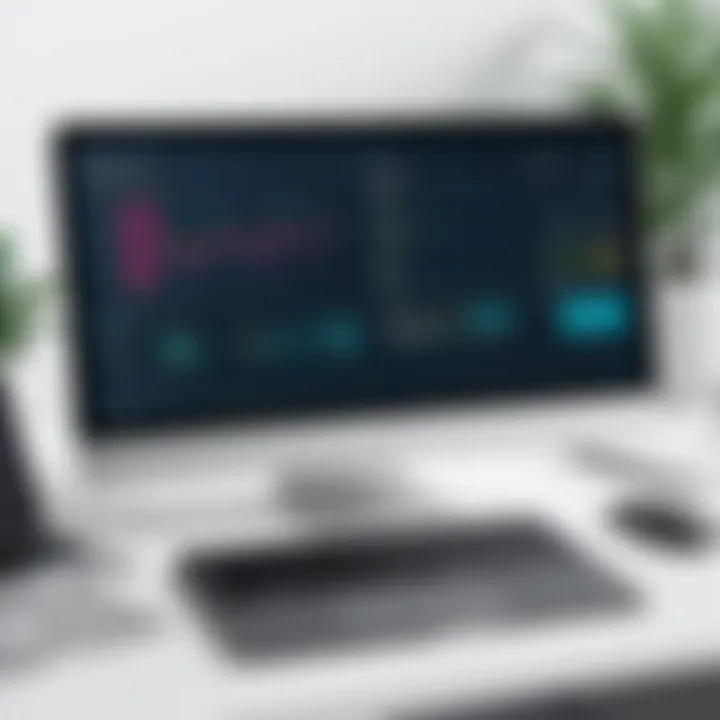
Key aspects of customizing outputs in SPSS include:
- Graphical Adjustments: Change visuals to improve clarity, like adjusting axis labels or data point colors.
- Table Modifications: Rearrange and format tables for readability by altering cell borders and font styles.
- Export Options: Save customized outputs in various file formats, such as PDF, Excel, or HTML, allowing for easy sharing and distribution.
In summary, the advanced features of SPSS, particularly the Syntax Editor and output customization tools, provide users with significant advantages in their data analysis processes. Mastering these functions can lead to more sophisticated results and increased productivity in analytical tasks.
Common Challenges and Solutions
In the realm of data analysis using SPSS, professionals often encounter various challenges that can hinder their workflow and efficiency. Understanding these common challenges and devising effective solutions is crucial for maximizing the capabilities of SPSS. This section focuses on two prevalent issues: data import challenges and error messages that may arise during the usage of the software. By addressing these elements, users can not only streamline their data analysis process but also mitigate frustrations typically associated with software limitations.
Data Import Issues
Data import is a foundational step in any analytical project. The inability to correctly import data can derail an analysis right from the start. SPSS supports a variety of formats such as Excel, CSV, and others, but each format presents its own set of challenges.
One frequent issue arises from data formatting. If the original data file contains errors or incompatible formats, SPSS may not be able to import the data as intended. For instance, numeric values formatted as text may prevent essential statistical calculations. It is advisable to review data files prior to importing them into SPSS. This ensures that all fields are correctly formatted and devoid of errors.
Another challenge is related to character encoding. Special characters can sometimes lead to problems. To solve this, users should ensure that the data is saved in a universally accepted format like UTF-8 before importing.
Here are a few tips to handle data import issues:
- Check file format compatibility: Ensure the data file is saved in a supported format.
- Review for hidden characters: Inspect for any invisible formatting issues that could disrupt the import.
- Utilize SPSS syntax: For advanced users, utilizing the SPSS syntax editor can provide more control over the import process.
Error Messages and Troubleshooting
Error messages in SPSS can be perplexing and frustrating for users. These messages often indicate that something is wrong, but they may not always clearly specify the source of the problem. Understanding common error messages can save time and help in troubleshooting effectively.
Common errors include those associated with variable definitions. An example is when a user tries to execute a command without defining the necessary variables beforehand. The error usually indicates a missing variable, which can easily be resolved by ensuring all variables are correctly defined in SPSS before running analyses.
Moreover, SPSS users might encounter errors during statistical tests due to non-conformity in data assumptions. For instance, running a t-test when the data does not meet the assumption of normality can lead to misleading results. Conducting preliminary tests for assumptions can help avoid such situations.
Here are effective troubleshooting steps for addressing error messages:
- Read error descriptions carefully: Many error messages contain clues for resolving issues.
- Consult the SPSS documentation: Reference the SPSS manual or online help for specific situations.
- Seek assistance from user forums: Communities like Reddit have users who may have faced similar problems and can offer solutions.
"Understanding the common challenges in SPSS enables users to elevate their analysis and confidently harness the software's capabilities."
Best Practices for Using SPSS
Using SPSS effectively is critical for accurate data analysis. This section focuses on best practices that enhance the user experience and output results. Proper utilization of SPSS can significantly improve the quality of research findings. For both novice and seasoned users, adhering to best practices fosters systematic and efficient analyses. They help in avoiding common pitfalls and streamline the overall process, leading to reliable and replicable results.
Data Cleaning Procedures
Data cleaning is one of the most important steps when working with SPSS. It involves identifying and correcting (or removing) inaccurate records from a dataset. The goal is to improve the quality of data before analysis.
Here are some essential practices for data cleaning in SPSS:
- Check for Missing Values: Missing values can distort statistical analyses. SPSS offers several techniques for handling these, such as replacements with mean or median values.
- Identify Duplicates: Duplicates can skew results. Utilize the "Sort Cases" and "Remove Duplicates" functions to ensure unique entries.
- Correct Data Entry Errors: Manual data entry often introduces errors. Regularly review the data for logical inconsistencies and typographical mistakes.
- Standardize Formats: Ensure consistency in data formats, particularly for categorical variables. For instance, choose between uppercase and lowercase consistently.
Using data cleaning procedures enhances the integrity of the dataset and enables the generation of valid results.
Documenting the Analysis Process
Documentation is vital for reproducibility and clarity in any statistical analysis. Properly documenting the analysis process in SPSS ensures that the steps taken are easily understandable for anyone reviewing the project. This is especially important in collaborative environments.
Key elements to incorporate include:
- Save Syntax: SPSS allows users to create syntax for their procedures. Save the syntax along with the output files to document the steps taken.
- Commenting: Use comments within the syntax to provide context about the purpose of each section. This is helpful for future reference or when updating analyses.
- Version Control: Keep track of changes made in SPSS files. Naming conventions or version logs can help identify the most relevant analysis version.
- Output Files: Save output files after each major analysis step. This segmentation helps locate specific findings easily later.
Effective documentation practices not only aid in validation and transparency but also enhance collaboration among team members, thereby enriching the analytical outcomes.
Ending
The conclusion serves as a critical element in solidifying the insights gained throughout this article on SPSS. It enables readers to reflect on the key points discussed and understand the broader implications of using SPSS in data analysis. Summarizing the information presented ensures that the essential aspects of the software are encapsulated, allowing users to grasp the core functionalities and applications of SPSS more effectively.
Summarizing Key Insights
In this article, we explored the various dimensions of SPSS, an indispensable tool for data analysis. From understanding its statistical capabilities to its user-friendly interface, the discussion aimed to highlight how SPSS can enhance research efficiency across fields such as social science, market research, and healthcare.
Key insights include the following:
- Comprehensive Statistical Analysis: SPSS allows robust statistical evaluation, offering users the tools for descriptive, inferential, and regression analysis.
- Effective Data Management: The software is designed to streamline data handling processes, making it easier for users to import, clean, and manipulate data sets.
- Helpful Visualization Tools: Graphical representation of data assists users in making sense of complex statistics, supporting more informed decision-making.
The adeptness of SPSS in addressing common data-related challenges, such as data import errors and troubleshooting erratic messages, enhances its appeal to both novices and seasoned professionals.
Future Developments in SPSS
Looking ahead, the evolution of SPSS promises to integrate more advanced features that align with the rapidly changing landscape of data analysis techniques. Innovations in machine learning and artificial intelligence are likely to permeate the SPSS framework, making predictive modeling more accessible and efficient.
Additionally, increased emphasis on cloud-based solutions may allow for better collaboration across teams and easier access to large data sets from multiple devices. This transition can improve real-time data analysis and expedite research outcomes.
Furthermore, user feedback will play a pivotal role in determining future updates and enhancements. Incorporating user suggestions is essential to maintain SPSS as a relevant and effective solution for diverse data analysis needs.
\n> "The future of SPSS looks promising as it embraces technological advancements that enhance analytical capabilities."
In summary, the conclusion emphasizes the importance of SPSS not only as a powerful tool but also as an evolving solution in the realm of data analysis. As we reflect on its relevance today, continuous adaptation and updates will ensure SPSS remains a frontrunner in meeting the ever-changing demands of data professionals.